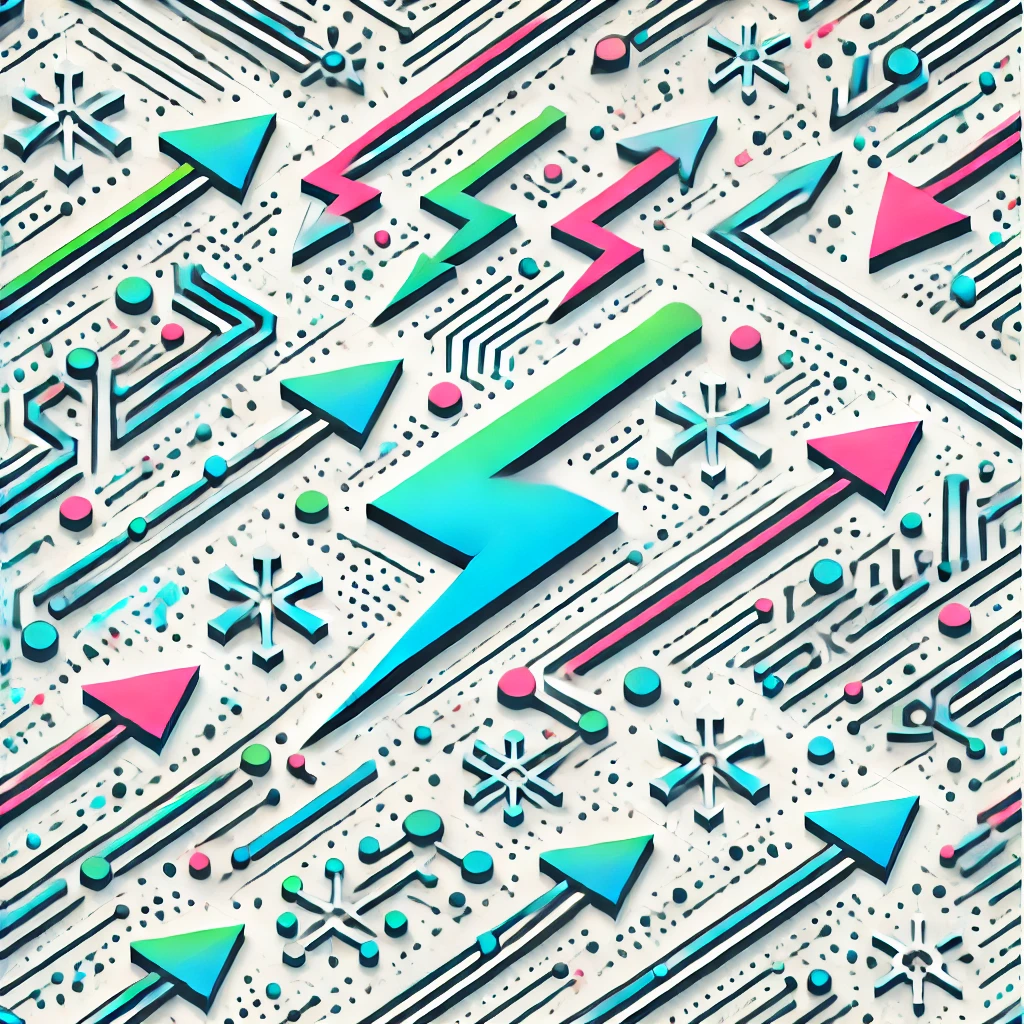
Central Limit Theorem
The Central Limit Theorem (CLT) states that when you take a large number of samples from any population, regardless of its distribution, the average of those samples will form a normal distribution, or bell curve, around the true population average. This occurs as the sample size increases, typically becoming noticeable with around 30 samples. The CLT is crucial in statistics because it allows us to make inferences about a population using sample data, ensuring that many statistical methods are reliable. It highlights the power of averages and the consistency of results across various scenarios.
Additional Insights
-
The Central Limit Theorem states that when you take a large enough sample from any population, regardless of its distribution, the average (or mean) of those samples will be roughly normally distributed, resembling a bell curve. This means that as you collect more data, the sample averages will cluster around the true average of the entire population, making predictions and statistical analysis more reliable. It allows researchers to use normal distribution techniques in various fields, even when the original data is not normally distributed, simplifying many real-world problems.
-
The Central Limit Theorem states that when you take a large enough sample from any population, regardless of its shape, the average of those samples will form a normal distribution (bell curve) around the true population average. This holds true even if the original data is not normally distributed. Essentially, this theorem allows us to make predictions about population parameters through sample statistics, making it a cornerstone of statistics. It underlines why many processes and phenomena in nature tend to follow patterns we can quantify and analyze.