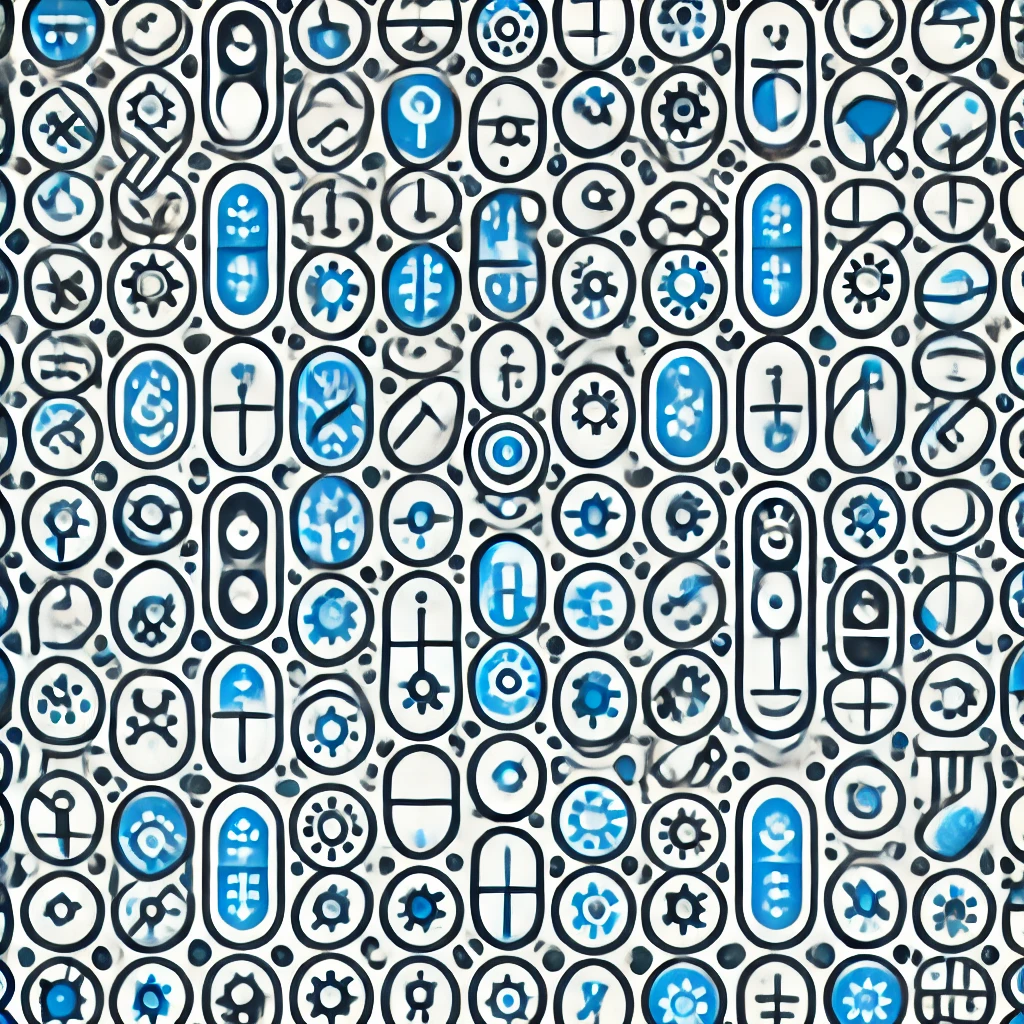
Causal Inference
Causal inference is the process of determining whether one event causes another, rather than merely being correlated. In philosophy, it explores how probabilities relate to possible worlds, considering what might have happened under different circumstances (counterfactuals). In statistics, it uses methods like experiments and observational studies to uncover causal relationships. Essentially, causal inference aims to provide clarity on how and why changes occur, helping us make informed decisions based on evidence. It acknowledges the complexity of real-world interactions while striving for a more precise understanding of cause and effect.
Additional Insights
-
Causal inference is the process of determining whether one event or action directly influences another. For example, if we want to know if exercising regularly leads to better health, causal inference uses data and statistical methods to establish a clear link between the two. It goes beyond mere correlation, which only shows that two things happen together but doesn't prove one causes the other. By controlling for other factors and using experimental or observational studies, researchers can draw more reliable conclusions about cause-and-effect relationships, helping us understand how changes in one area might impact another.
-
Causal inference is the process of determining whether one event or action directly causes another. It goes beyond mere correlation, which can show that two things occur together but doesn’t explain if one actually influences the other. For example, if we observe that people who exercise regularly tend to be healthier, causal inference helps us assess whether exercise is the reason for improved health or if other factors might be at play. Researchers use various methods, like experiments or statistical modeling, to establish causal relationships and understand how different factors interact in real-world situations.