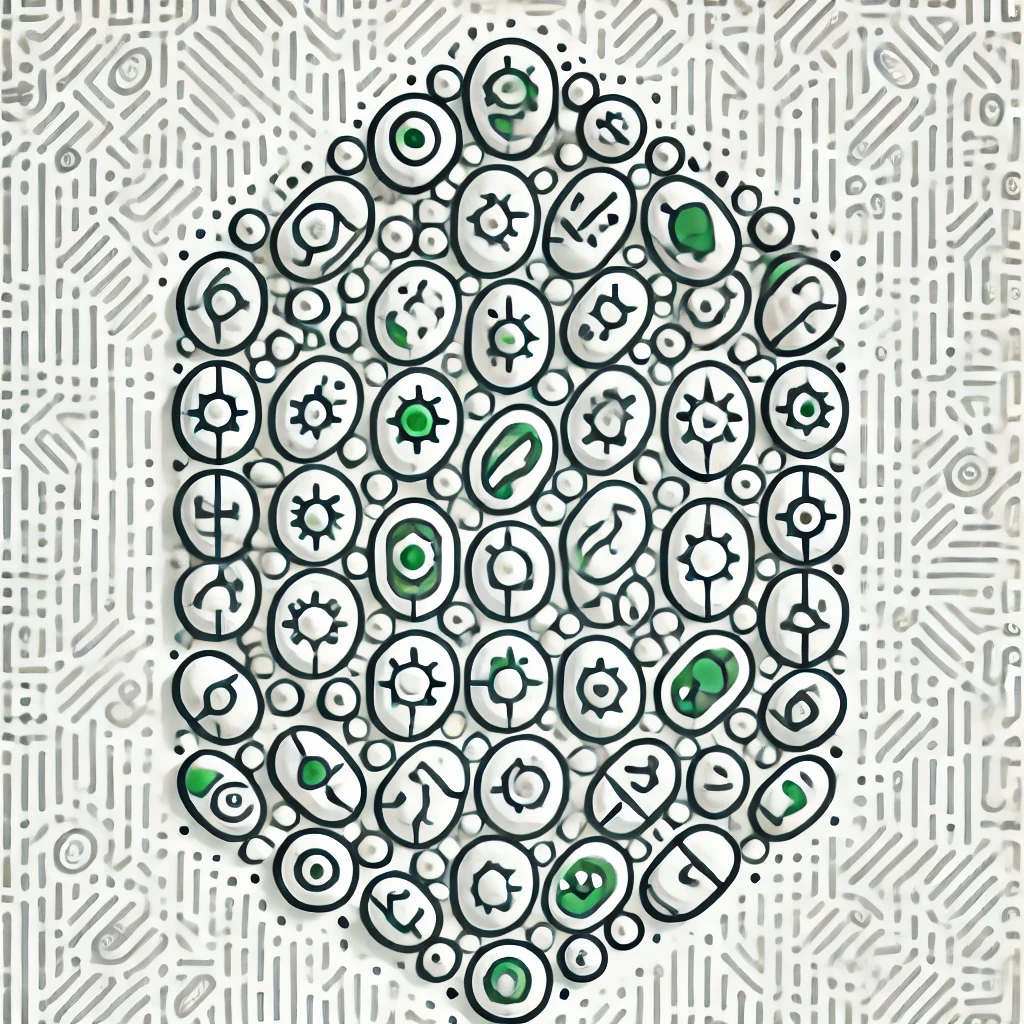
Probabilistic Graphical Models
Probabilistic Graphical Models (PGMs) are a way to represent complex relationships between variables using graphs. In these models, nodes represent random variables, while edges between them show how these variables influence each other. This framework allows us to understand uncertainties and make predictions by applying probability theory. PGMs are widely used in fields like AI, statistics, and machine learning, enabling tasks such as decision-making, diagnosing problems, and recognizing patterns by efficiently capturing and analyzing relationships among uncertain data.
Additional Insights
-
Probabilistic graphical models are a way to represent complex relationships among uncertain variables using graphs. In these models, nodes represent variables, while edges (connections) indicate dependencies between them. They help us understand how changes in one variable can affect others, enabling us to make predictions or decisions in uncertain situations. Common applications include natural language processing, computer vision, and medical diagnosis, where understanding intricate relationships and uncertainties is crucial. Essentially, they provide a structured method to visualize and calculate probabilities, making complex data more manageable and interpretable.