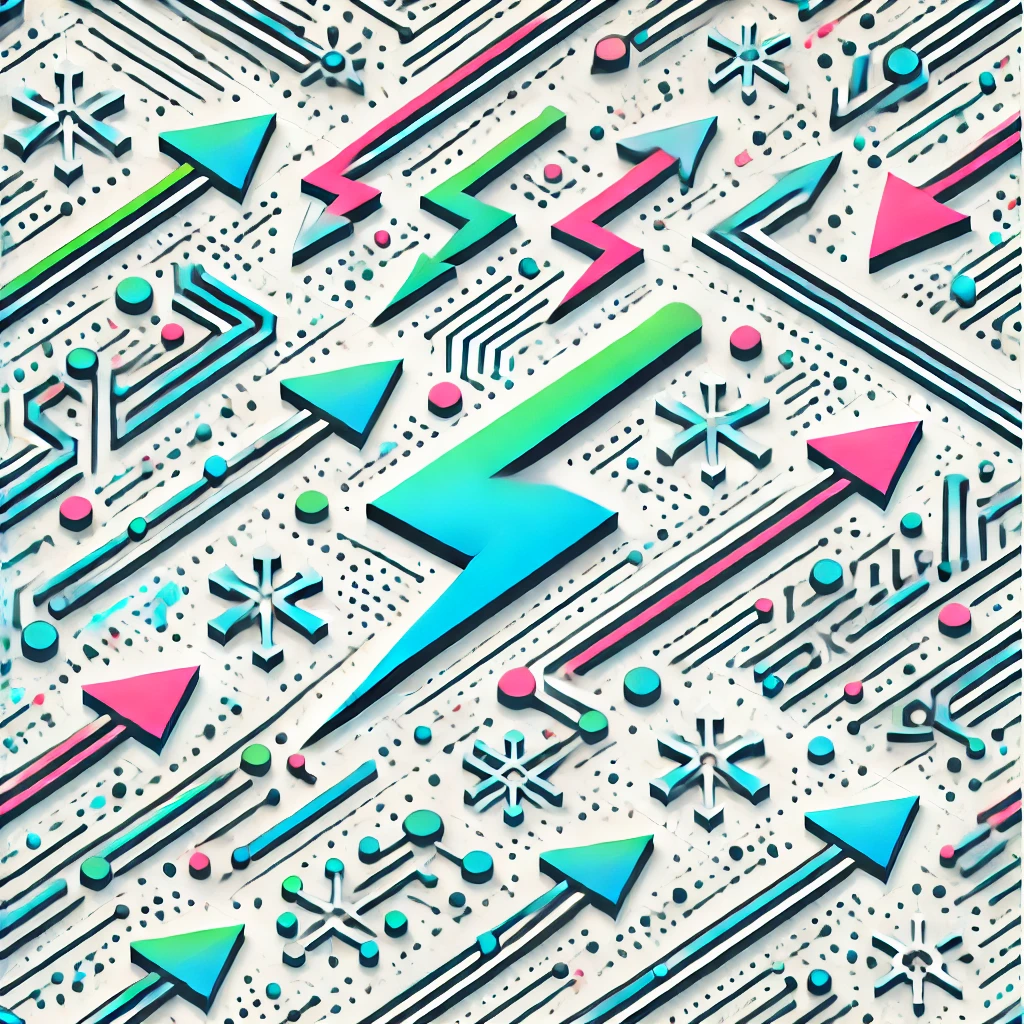
F1 Score
The F1 Score is a measure used to evaluate the performance of a classification model, balancing two important metrics: precision and recall. Precision indicates how many of the predicted positive results were actually correct, while recall measures how many of the actual positive cases were identified. The F1 Score combines these two into a single number, ranging from 0 to 1, where 1 means perfect precision and recall. It is particularly useful when dealing with imbalanced datasets, where one category is much more common than the other, as it ensures a fair assessment of the model’s effectiveness.