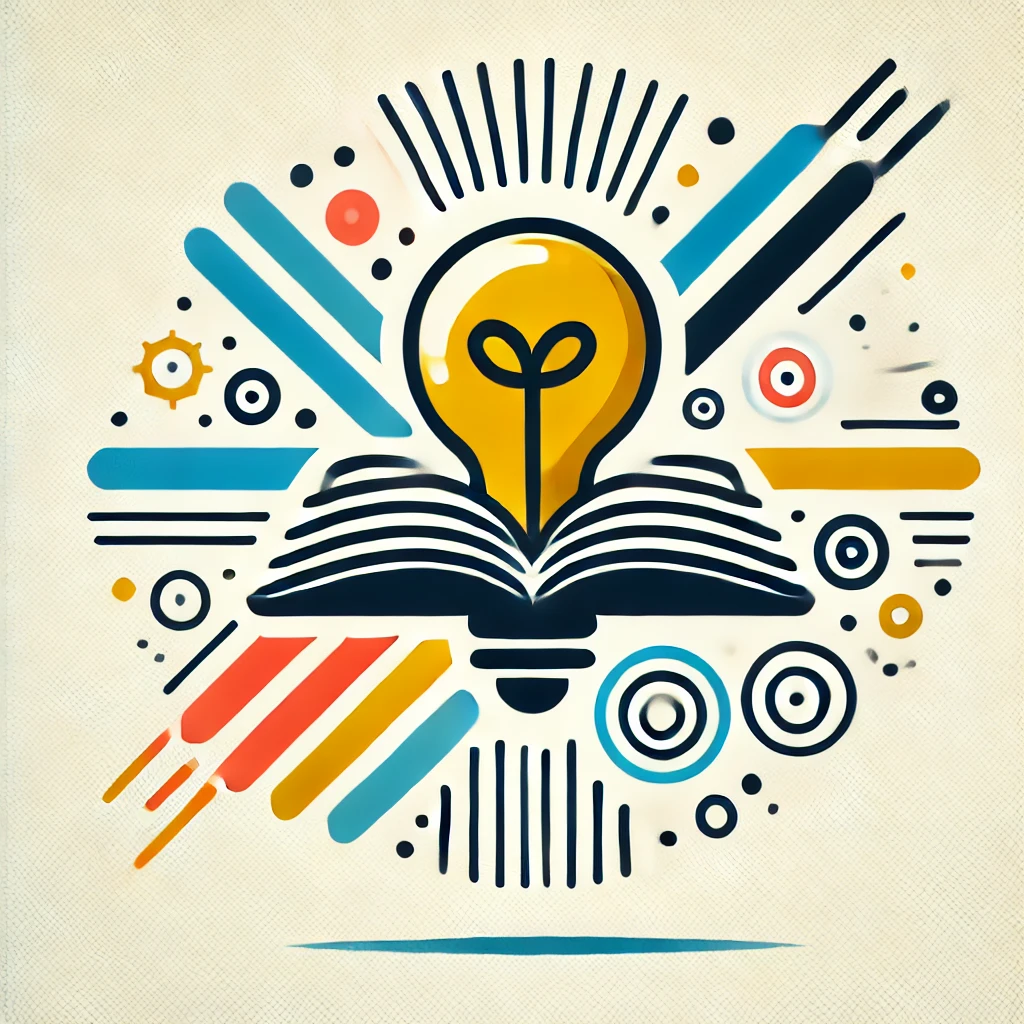
Confusion Matrix
A confusion matrix is a tool used to assess the performance of a classification model in machine learning. It presents a table that shows the number of correct and incorrect predictions made by the model, categorized into four outcomes: true positives (correctly identified), true negatives (correctly rejected), false positives (incorrectly labeled as positive), and false negatives (missed positives). By analyzing these results, we can better understand the model's strengths and weaknesses, leading to improvements in accuracy and reliability. This matrix helps in evaluating how well the model performs in different scenarios.
Additional Insights
-
A confusion matrix is a tool used to evaluate the performance of a classification model, like a quiz that categorizes answers into correct and incorrect. It displays four key outcomes: True Positives (correctly identified items), True Negatives (correctly rejected items), False Positives (wrongly identified items), and False Negatives (missed items). By organizing these outcomes in a matrix format, it helps visualize how well the model is performing, highlighting areas of accuracy and common mistakes. This allows for a better understanding of where improvements are needed.