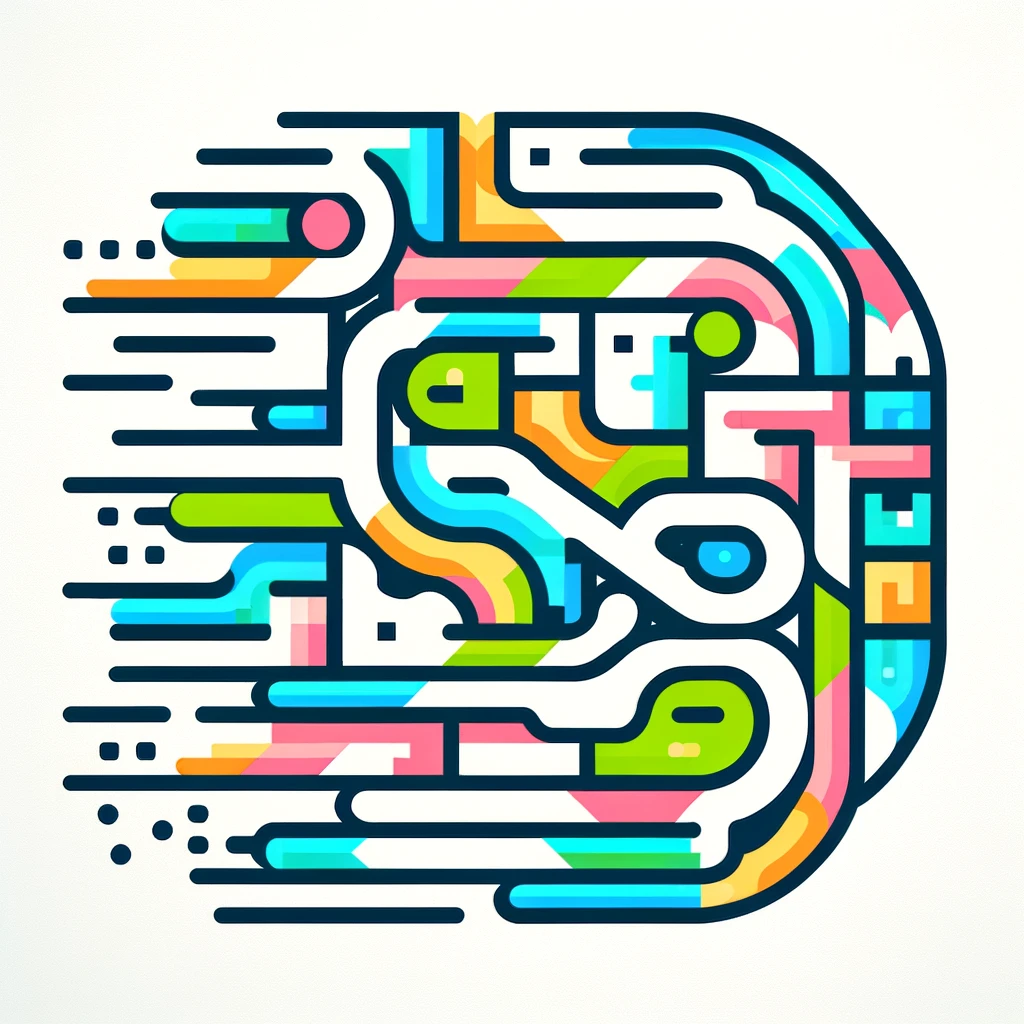
Tikhonov Regularization
Tikhonov Regularization is a mathematical technique used to solve problems where we have incomplete or noisy data. It adds a penalty to the solution to prevent overfitting, which occurs when a model learns too much from the noise in the data and loses generalizability. Essentially, Tikhonov Regularization balances fitting the data well with maintaining a simpler, more stable solution. This approach is commonly used in fields like statistics, machine learning, and image processing to improve the reliability of results when the data is uncertain or imperfect.