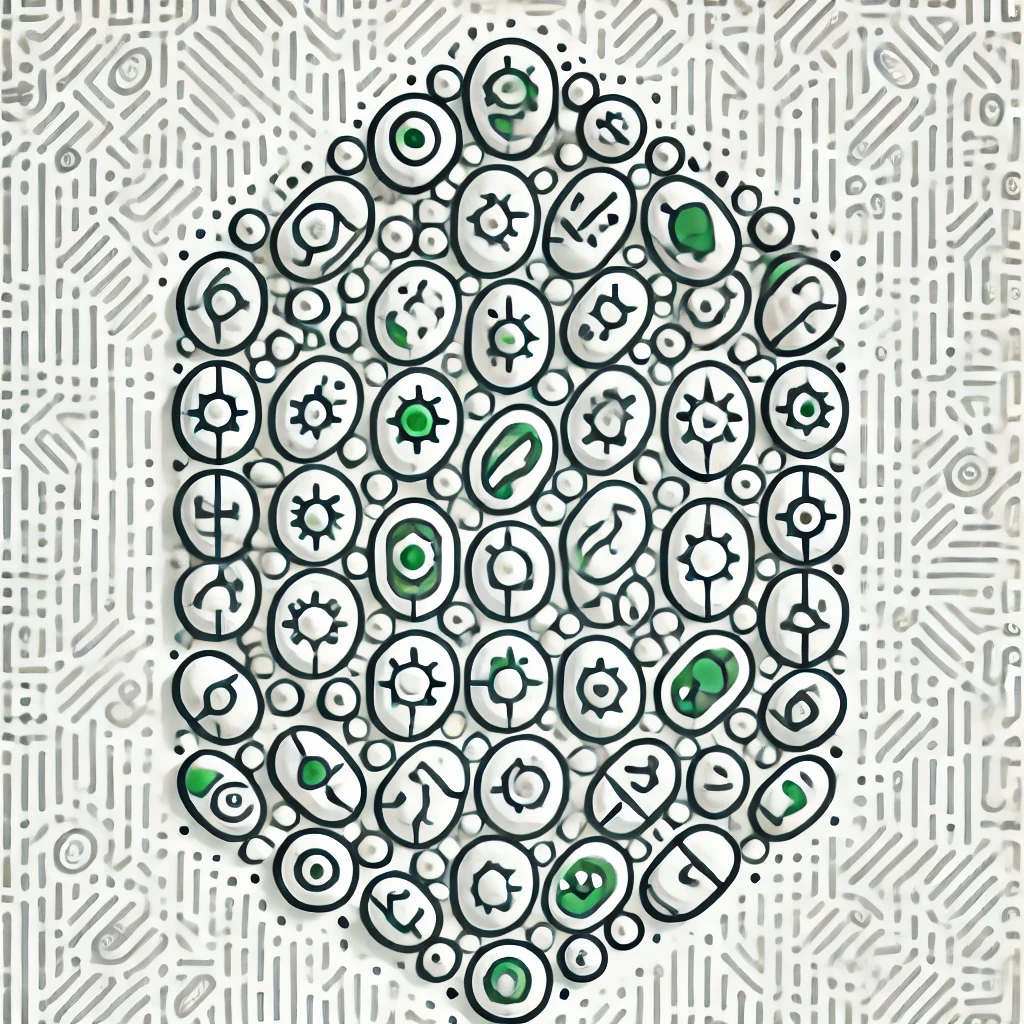
Regularization techniques
Regularization techniques are methods used in machine learning to prevent models from becoming too complex and overly fitting the training data, which can lead to poor performance on new, unseen data. Imagine trying to draw a curve that fits a set of points perfectly; while it may seem accurate, it can be erratic and miss the overall trend. Regularization encourages simpler models by adding a penalty for complexity, maintaining a balance between fitting the data well and keeping the model general enough to perform better in real-world scenarios. This helps improve the model's predictive power.