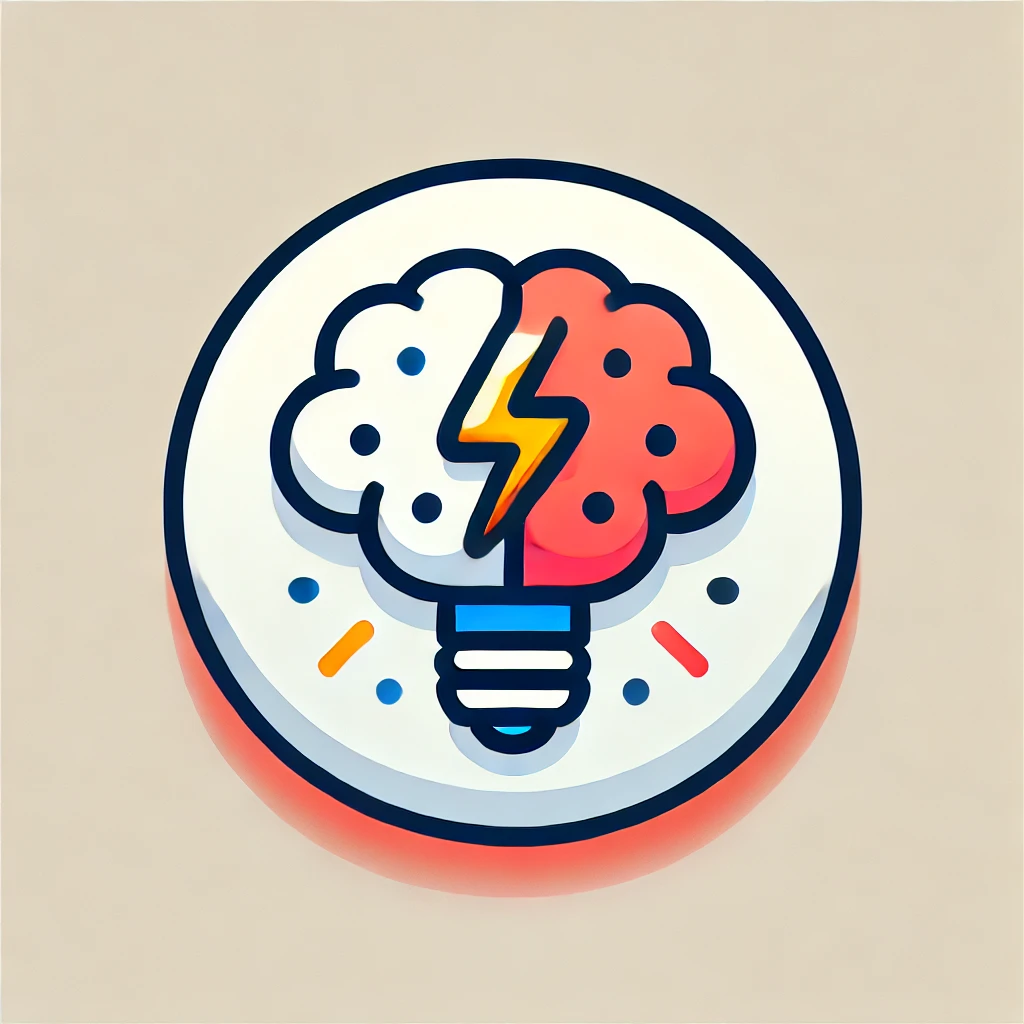
Regularization Methods
Regularization methods are techniques used in statistics and machine learning to prevent models from becoming too complex and overfitting the data they are trained on. Overfitting occurs when a model learns the noise in the data rather than the actual patterns, leading to poor performance on new data. Regularization adds constraints or penalties to the model, encouraging it to prioritize simplicity and generalization. Common types include Lasso and Ridge regularization, which adjust the importance of different model parameters. By promoting a balance between fitting the training data and maintaining simplicity, regularization helps improve model accuracy and reliability.