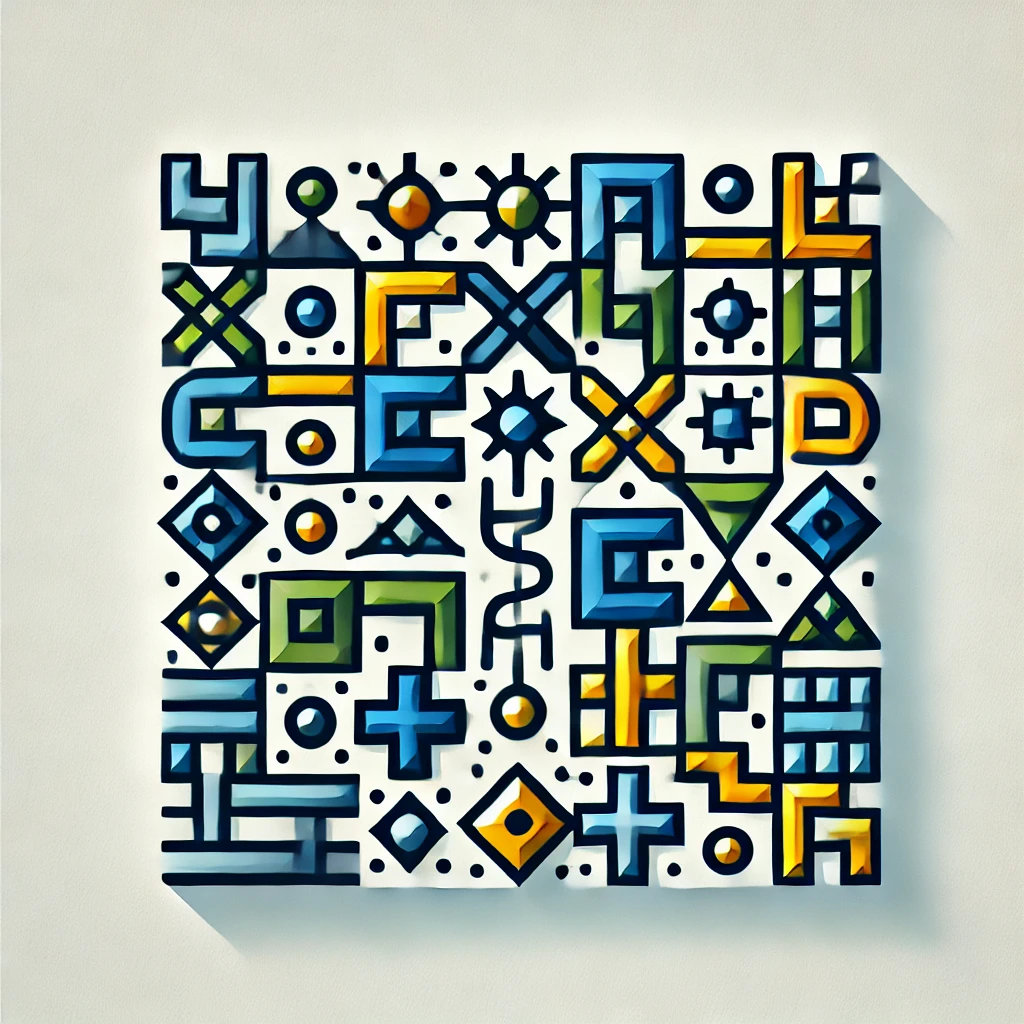
Jeffreys Prior
Jeffreys Prior is a statistical method used in Bayesian analysis to choose a starting point for estimating parameters when you have limited information. It is based on the principle of maximizing uncertainty, ensuring that the prior distribution reflects what you know or don't know about the parameters. Jeffreys Prior is particularly useful because it is invariant under reparameterization, meaning it remains consistent if the parameters are transformed. This approach helps researchers avoid biases that might arise from arbitrary choices and allows for a more objective analysis of data.