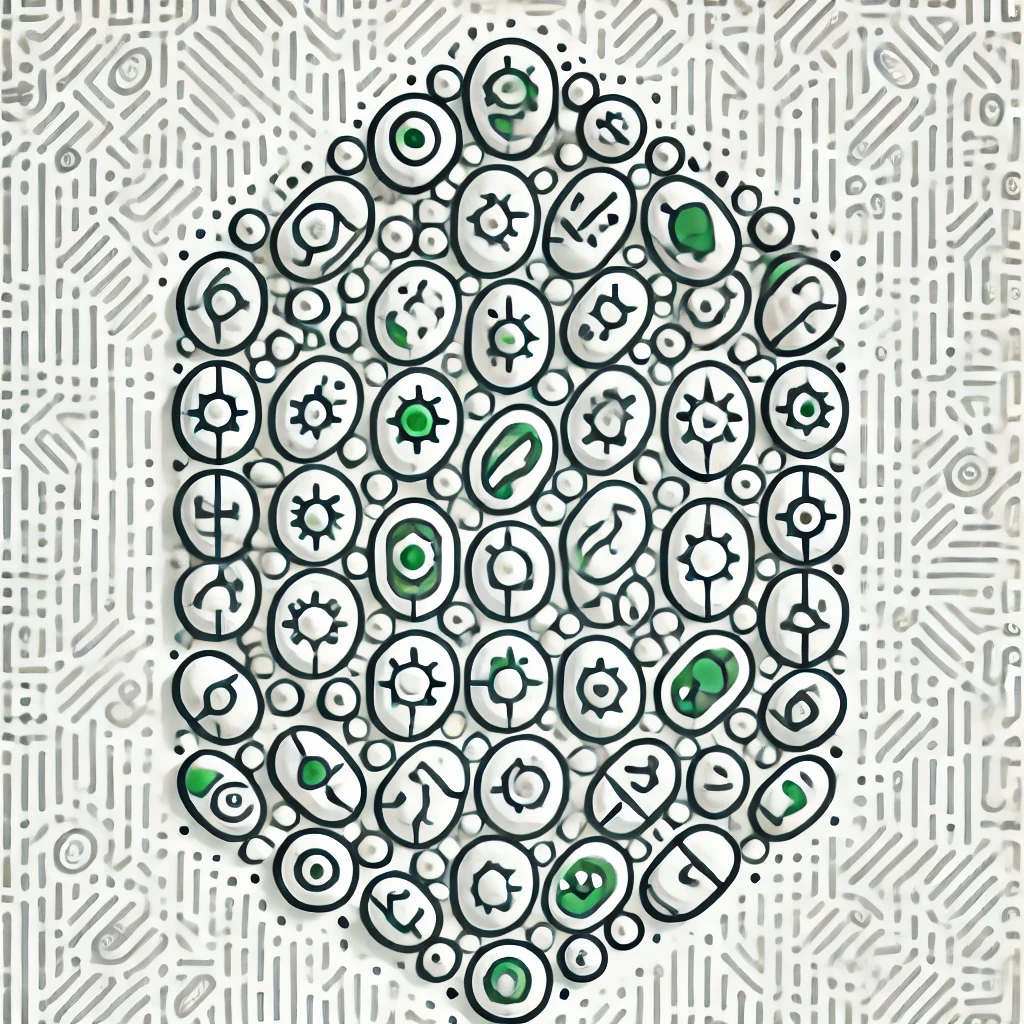
Jaynes' Maximum Entropy Principle
Jaynes' Maximum Entropy Principle is a method for making predictions or inferences based on limited information. It suggests that, when faced with uncertainty, we should select the probability distribution that is the least informative while still respecting the known constraints (like observed data). This approach allows us to derive conclusions that are as unbiased as possible. Essentially, it provides a way to make educated guesses by maximizing the uncertainty (entropy) about unknown factors, ensuring that our assumptions are not more specific than what the available information justifies.
Additional Insights
-
Jaynes' maximum entropy principle suggests that when we make predictions or infer probabilities about a system, we should use the most unbiased estimates possible, which means maximizing entropy. Entropy, in this context, measures uncertainty or randomness. So, when we have limited information, we should spread our probabilities as evenly as possible across all outcomes that are consistent with what we know, avoiding unwarranted assumptions. This approach helps ensure our conclusions are robust and reflect the uncertainty in our knowledge, leading to more reliable and fair predictions about uncertain events.