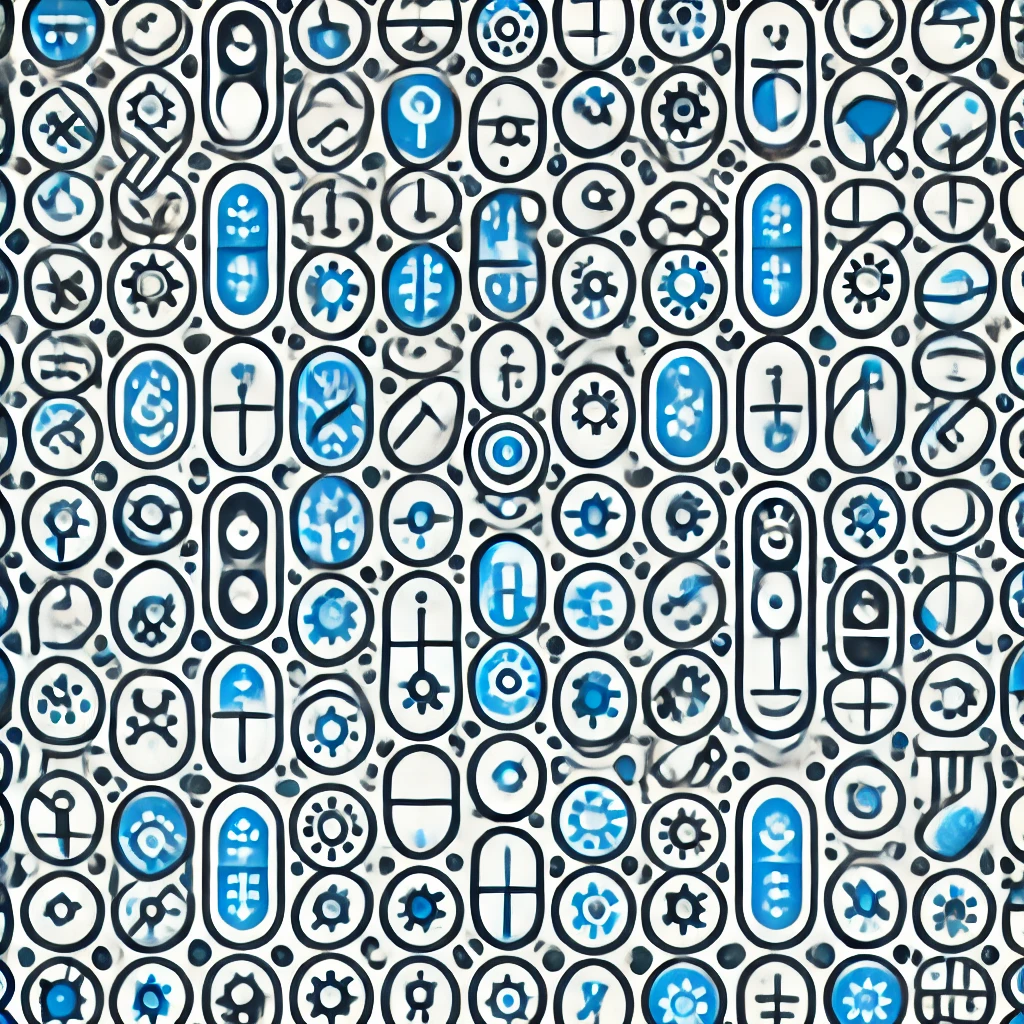
Gauss-Markov theorem
The Gauss-Markov theorem states that in a linear regression model, if certain conditions are met—like having a random sample and no perfect multicollinearity—the best, unbiased estimates of the unknown parameters can be found using ordinary least squares (OLS). “Best” means these estimates have the smallest possible variance among all linear estimators, ensuring they are the most precise. Essentially, this theorem assures us that under the right conditions, the OLS method provides reliable predictions with minimal error in the context of statistical modeling.
Additional Insights
-
The Gauss-Markov Theorem states that in a linear regression model, if the errors (differences between observed and predicted values) are unbiased, have constant variance, and are not correlated, then the Ordinary Least Squares (OLS) estimator produces the most accurate (minimum variance) estimates of the parameters. In simpler terms, if certain conditions are met, the way we usually calculate relationships in data gives us the best possible predictions. This theorem is fundamental in statistics, as it assures the reliability of estimates made through this common method.