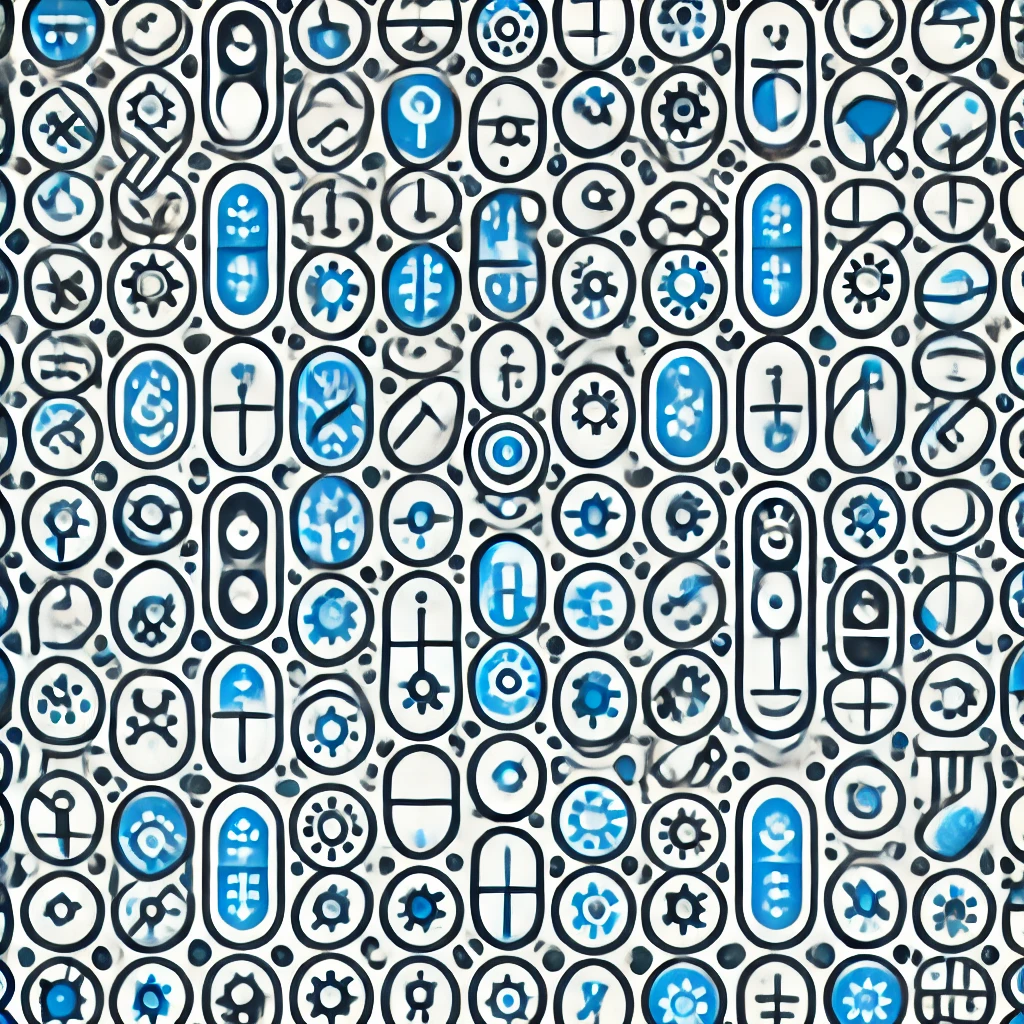
Smoothing Techniques
Smoothing techniques are methods used to reduce noise and fluctuations in data. Imagine a bumpy road; smoothing helps to create a smoother path. In general knowledge, this could apply to trends in statistics or data analysis. By using smoothing, we can identify underlying patterns more clearly without being distracted by random variations. Techniques like moving averages or exponential smoothing adjust the data to highlight the bigger picture, making it easier to understand overall trends—like predicting weather patterns or stock market movements—while minimizing short-term anomalies.
Additional Insights
-
Smoothing techniques are methods used to reduce noise or fluctuations in data, making trends easier to identify. Imagine you’re trying to find patterns in a bumpy road; smoothing helps flatten the bumps for a clearer view. Common examples include moving averages, where you take the average of a set number of data points over time, and exponential smoothing, which gives more weight to recent observations. These techniques are widely used in fields like finance, weather forecasting, and data analysis to make predictions and inform decisions based on clearer insights rather than erratic data points.