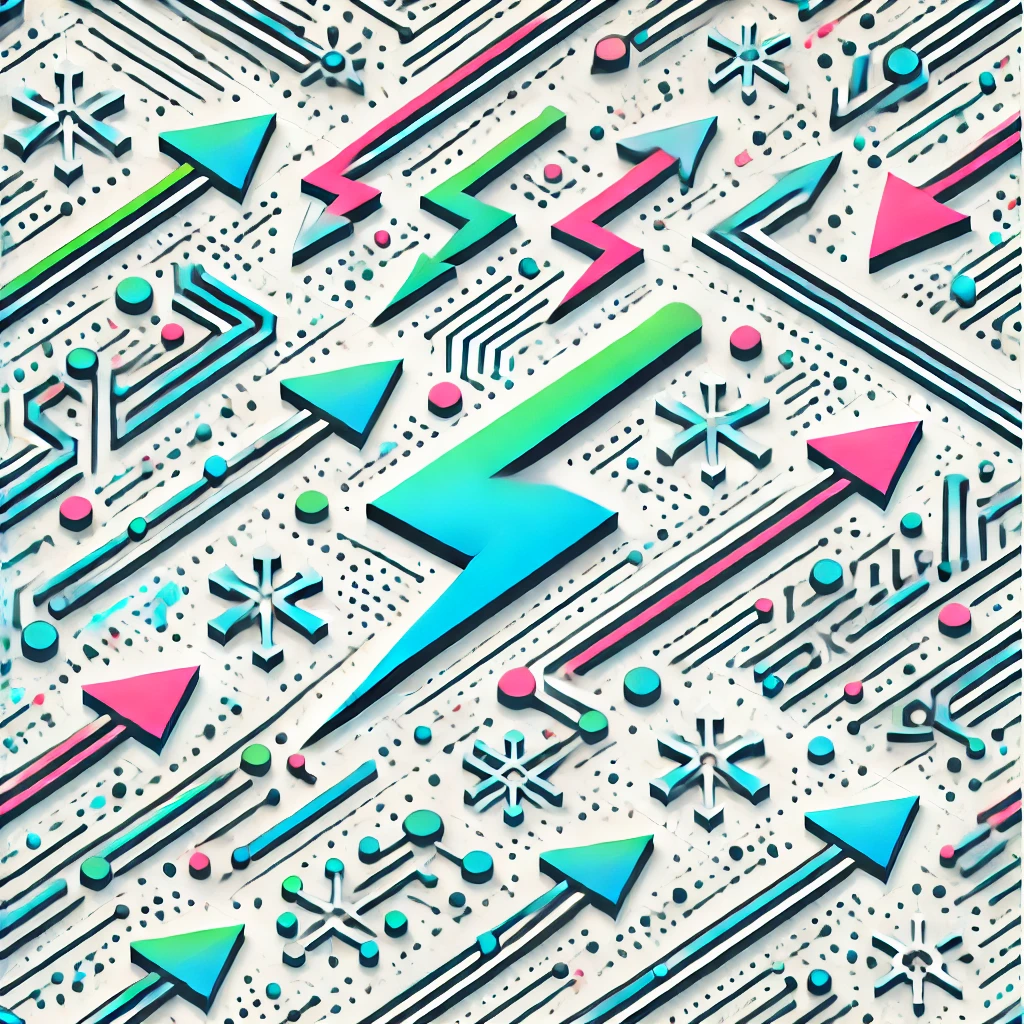
Residual Learning
Residual Learning is a concept used in deep learning, particularly in neural networks. It simplifies the training process by creating shortcuts for data to skip layers. Imagine trying to solve a complex puzzle; sometimes, it’s easier to focus on what you can add or change, instead of starting from scratch. Residual Learning allows the model to learn the “residuals”—the small changes needed—rather than the entire output. This makes it easier for the network to adjust and improve its predictions, resulting in faster and more accurate learning, especially in deep architectures.