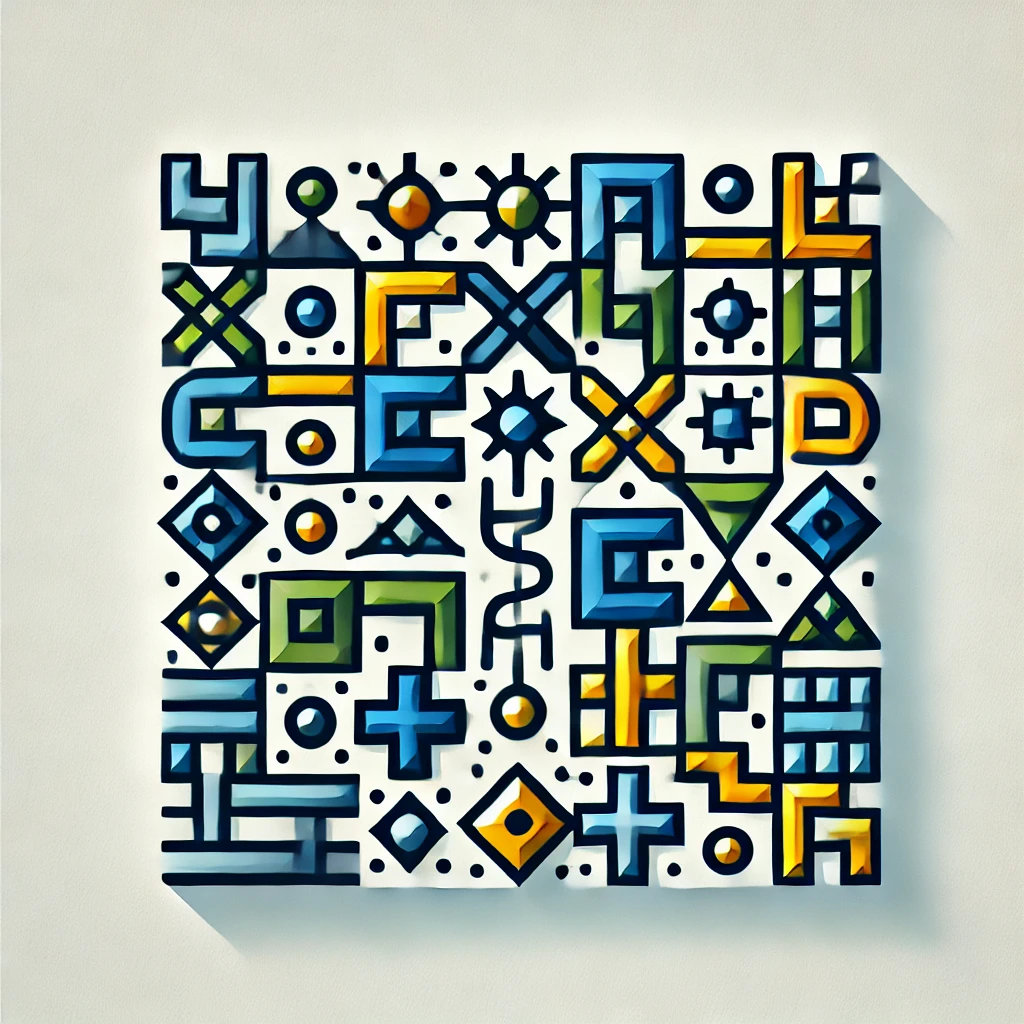
Residual Networks
Residual Networks (ResNets) are a type of deep learning model designed to improve training of very deep neural networks. They use "skip connections" that let information bypass some layers, allowing the network to learn residual functions—differences between the input and desired output—more effectively. This approach helps prevent problems like vanishing gradients, where signals get too weak to influence learning in deep layers. As a result, ResNets can be much deeper while maintaining accuracy, enabling them to recognize complex patterns in data such as images or speech more reliably.