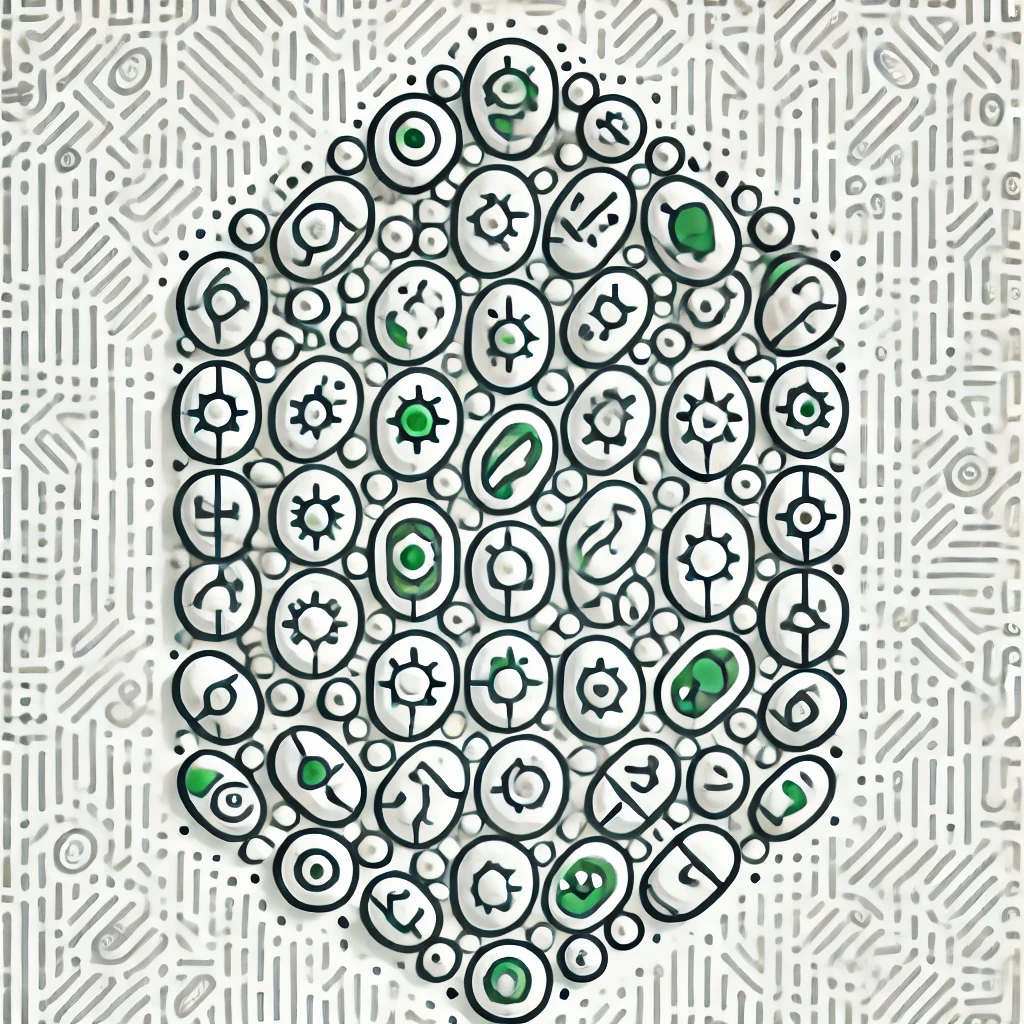
Matrix Concentration Inequalities
Matrix concentration inequalities are mathematical results that describe how a random matrix behaves in relation to its expected value. They quantify how much a random matrix can deviate from this expected value, similar to how concentration inequalities for numbers describe the likelihood that a random variable is far from its average. These inequalities are important in statistics, machine learning, and data science, as they help to understand the reliability of estimates and the performance of algorithms when dealing with large datasets or high-dimensional spaces. Essentially, they provide a way to assess the "regularity" of random matrices.