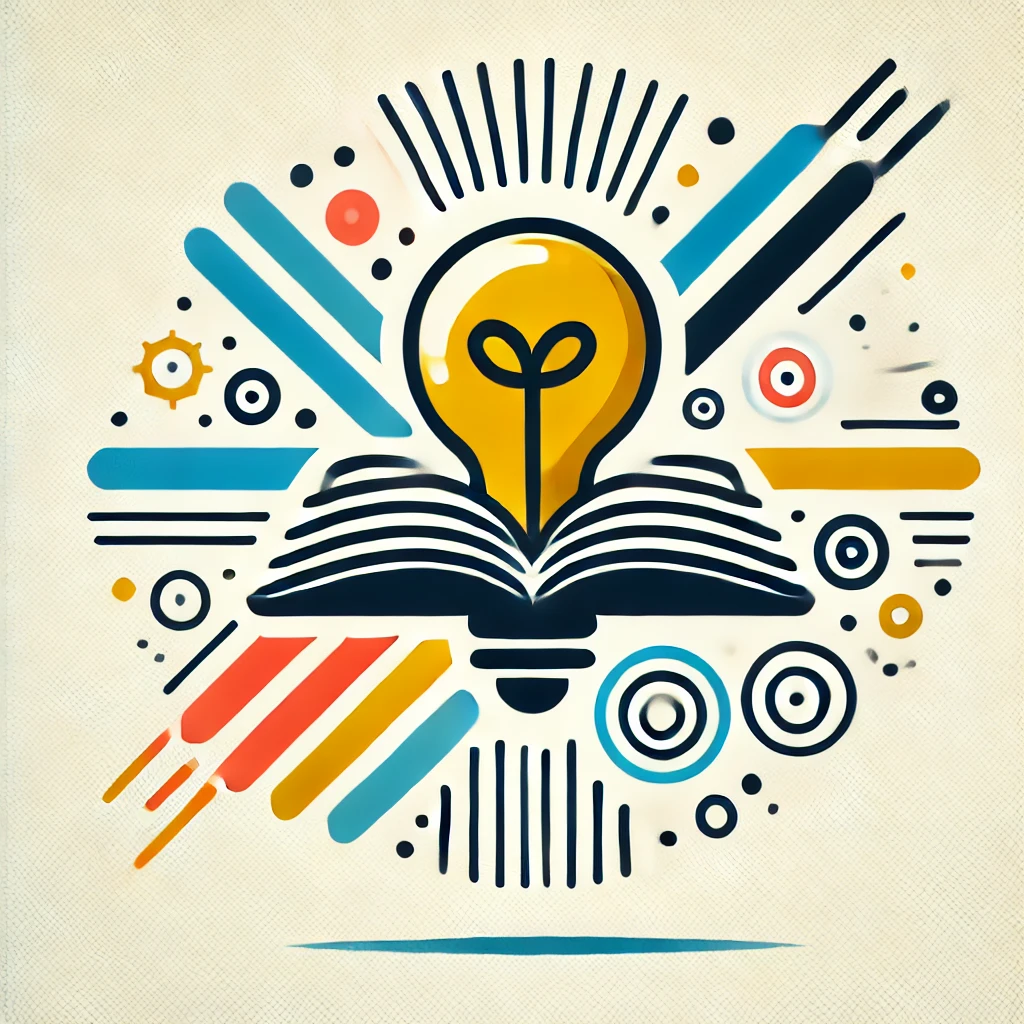
EM clustering
EM clustering, or Expectation-Maximization clustering, is a statistical technique used to group data into clusters based on similarities. It works in two main steps: first, it estimates the cluster membership for each data point (Expectation step), and then it updates the cluster parameters, like their shapes and centers, based on those memberships (Maximization step). This process repeats until the clusters stabilize. EM clustering is particularly useful when the data has underlying structures that are not immediately obvious, helping researchers and analysts to uncover patterns and insights in complex datasets.