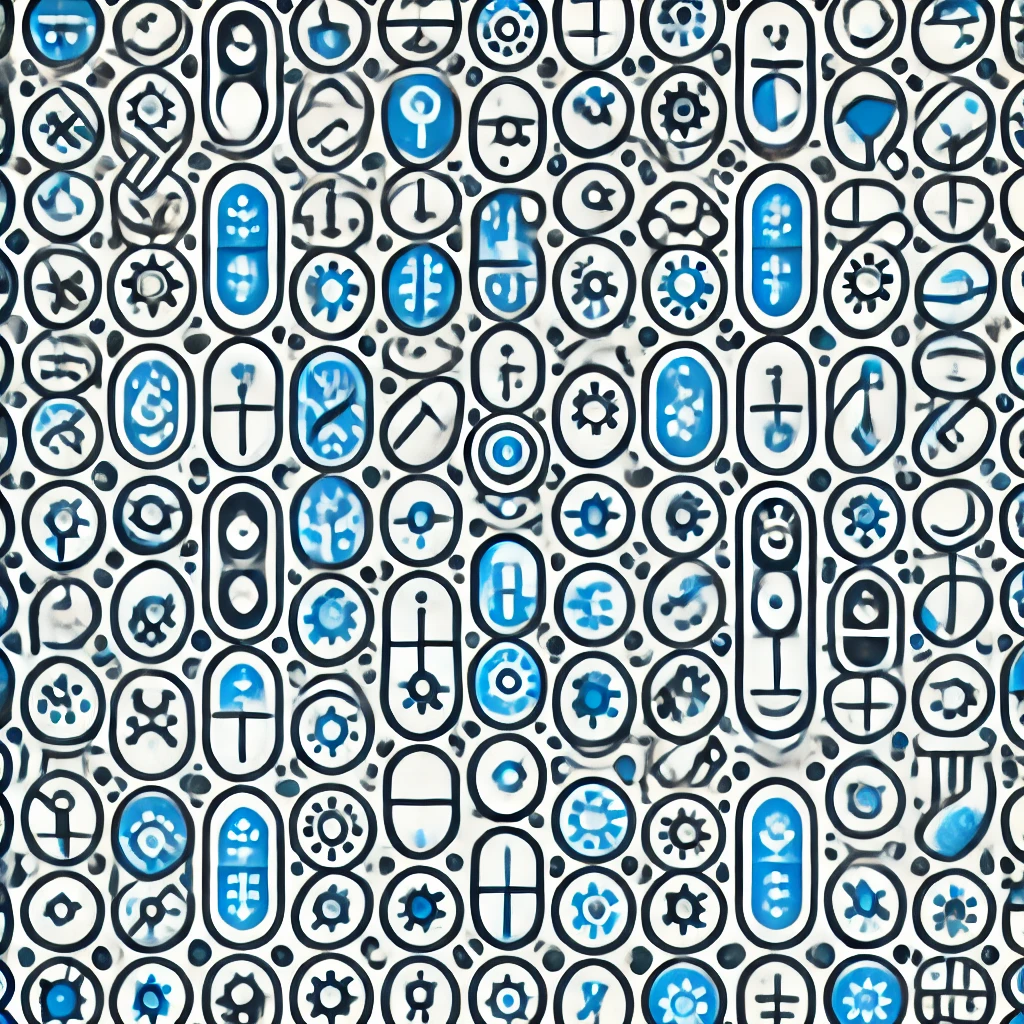
Privacy-preserving machine learning
Privacy-preserving machine learning refers to techniques that allow computers to learn from data while protecting individuals' private information. Instead of using personal data directly, methods like encryption, anonymization, or federated learning are employed. These techniques ensure that even though the machine learns patterns and insights from data, sensitive information remains secure and cannot be easily traced back to any individual. This approach balances the benefits of data-driven insights with the need to safeguard personal privacy, making it essential for applications in healthcare, finance, and beyond.