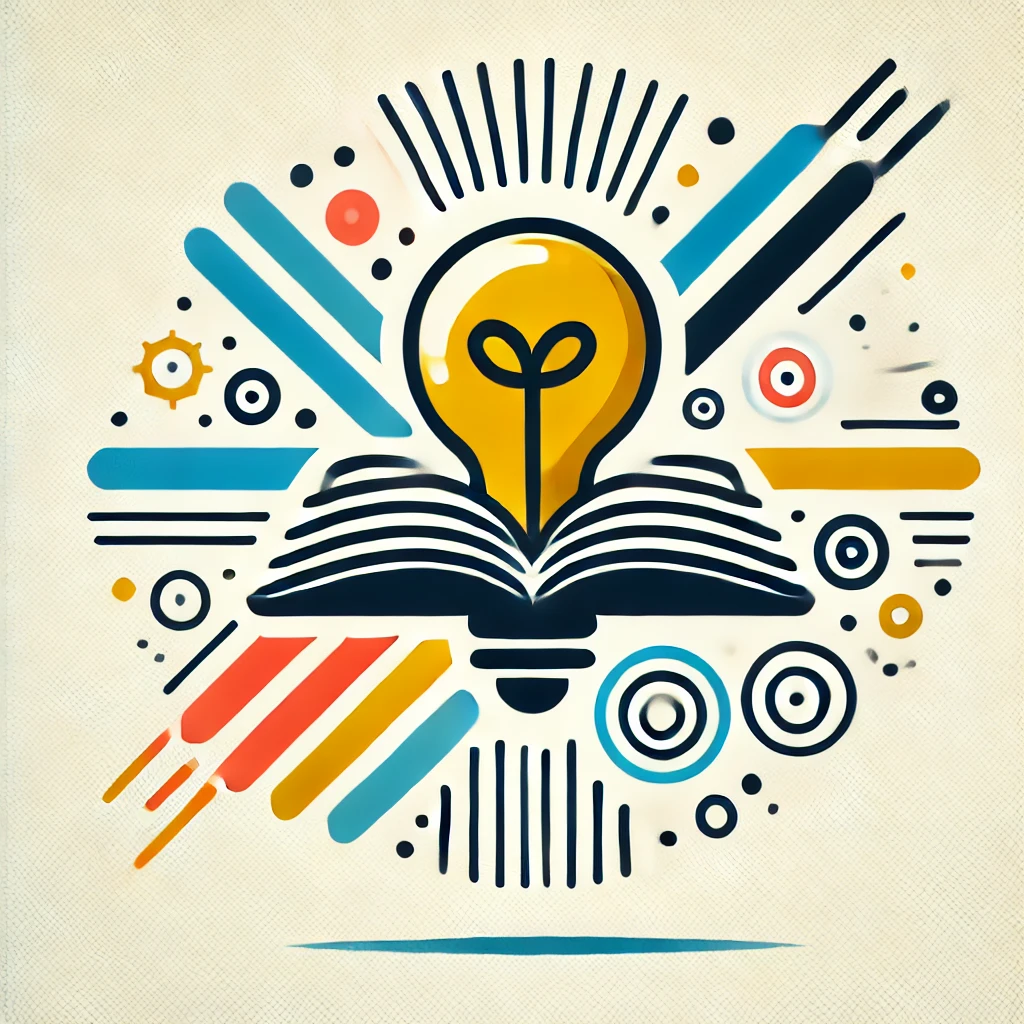
federated learning
Federated learning is a way to train machine learning models using data from many devices or locations without moving the data itself. Instead of collecting all data in one place, each device (like a smartphone) updates a shared model locally. These updates are then sent to a central server, which combines them to improve the overall model. This process preserves privacy because raw data stays on individual devices, reducing security risks. Federated learning enables collaborative AI development while respecting data confidentiality, making it useful for applications like personalized suggestions, medical research, and secure data analysis.