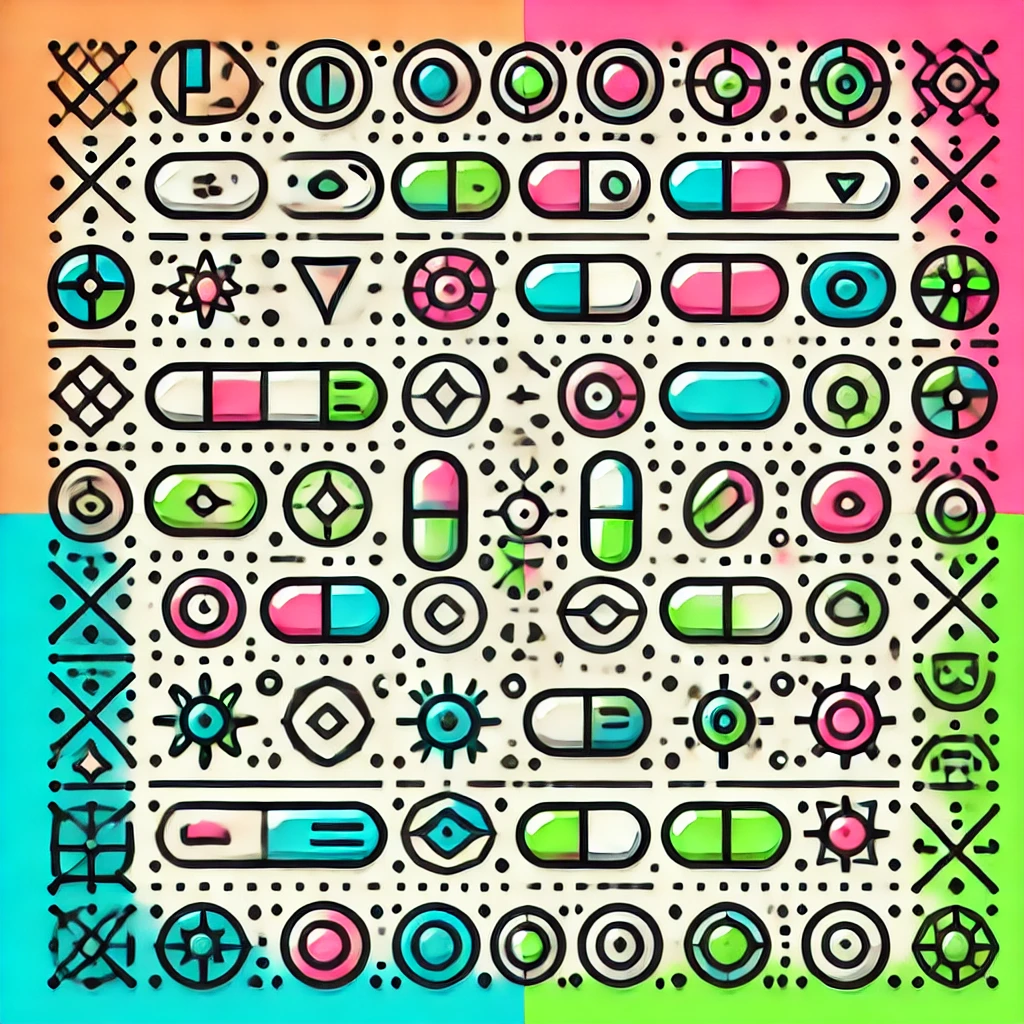
Yosinski et al. (2014)
Yosinski et al. (2014) studied how deep neural networks learn features at different stages. They found that early layers detect simple patterns like edges, while deeper layers identify complex features like objects. When changing or transferring parts of a trained network, the usefulness depends on how similar the tasks are; early features are generally more general, while later features are more task-specific. Their work helps us understand which parts of a neural network are universal versus specialized, guiding better design and transfer of deep learning models across different problems.