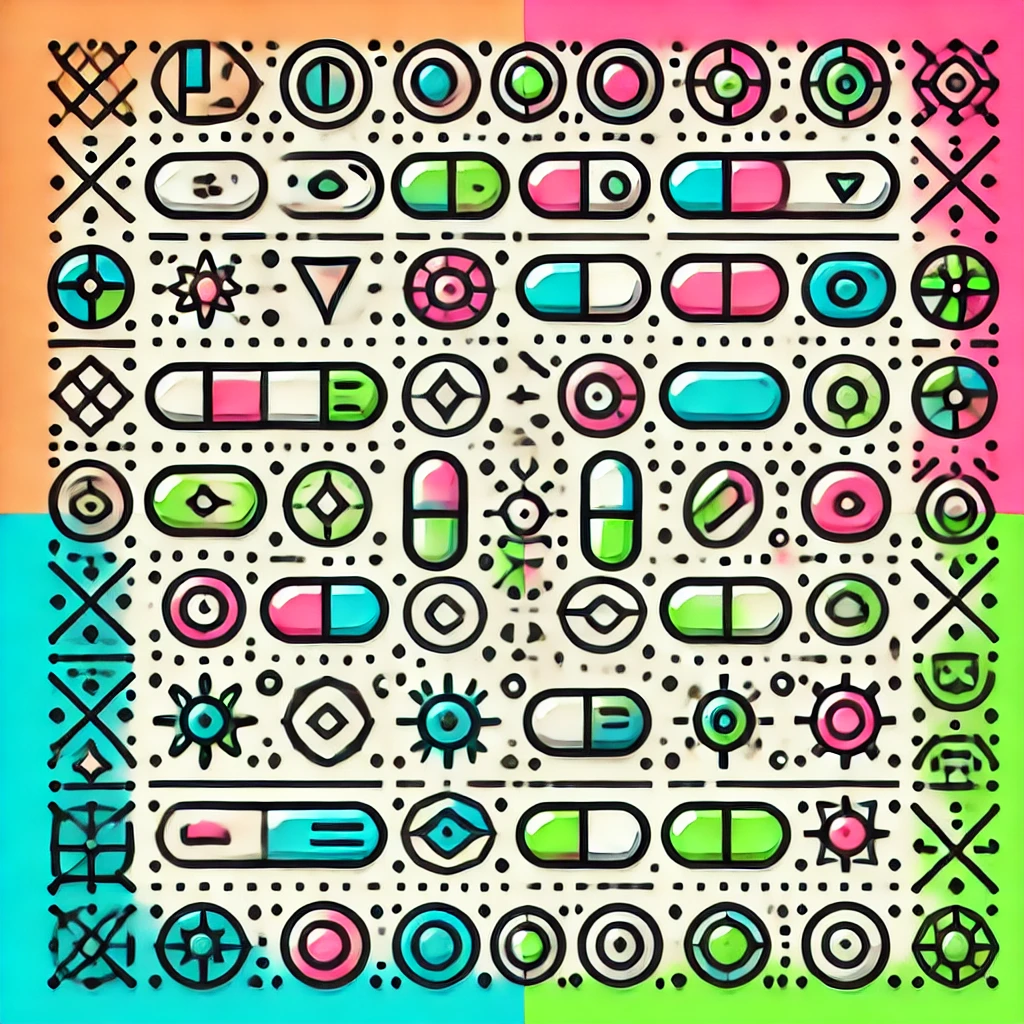
Support Vector Regression
Support Vector Regression (SVR) is a machine learning technique used to predict continuous outcomes by fitting a model to data points. It works by finding a smooth line (or curve) that best captures the pattern in the data while allowing for some errors within a specified margin. The goal is to minimize the errors while keeping the model as simple as possible, focusing on the most critical data points—called support vectors—that influence the fit. SVR is effective for modeling complex relationships and balancing accuracy with robustness in predictions.