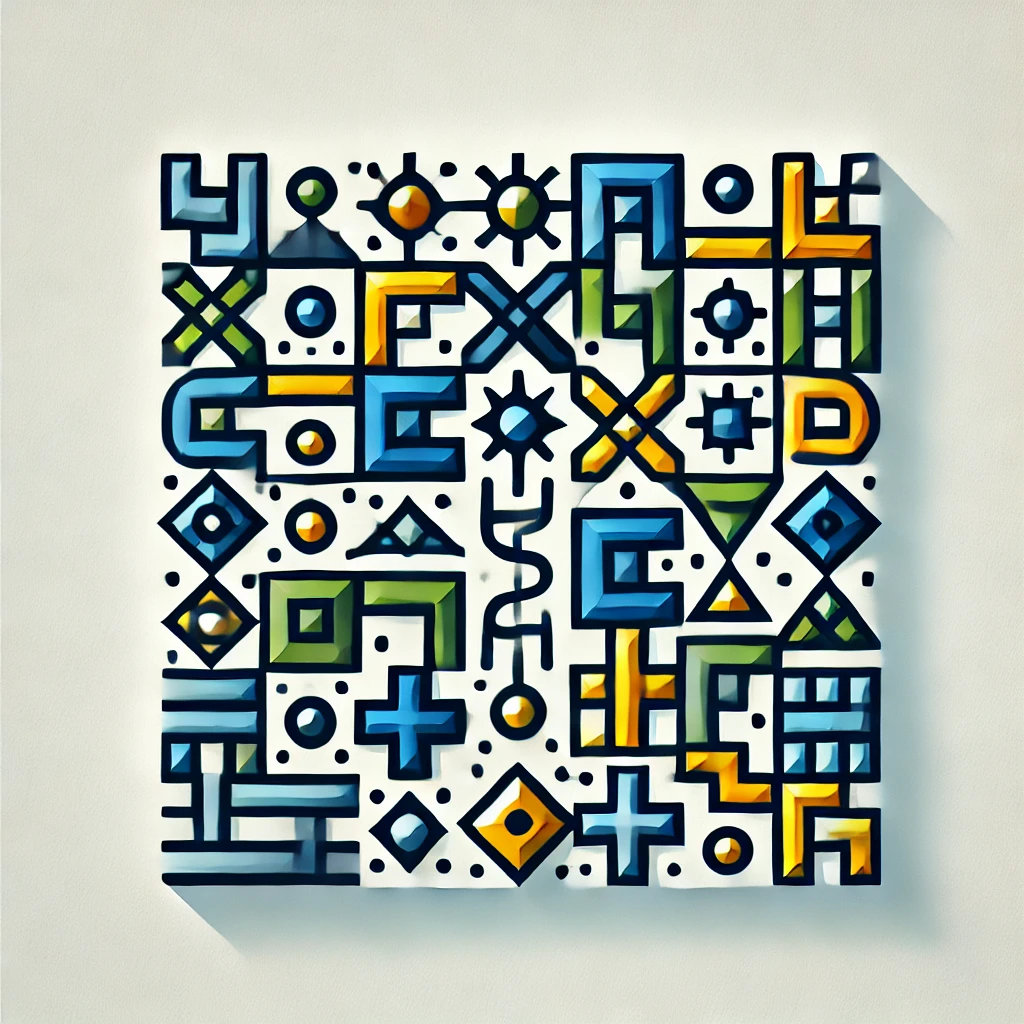
Squire's AleNone
Squire’s AleNone is a statistical method used in data analysis and machine learning to improve feature selection and model robustness. It works by randomly removing parts of the data or features during the training process, which helps prevent overfitting—where a model performs well on training data but poorly on new data. This technique encourages the model to focus on the most important, stable patterns, leading to better generalization. In essence, AleNone enhances the reliability and accuracy of predictive models by introducing controlled randomness during the learning process.