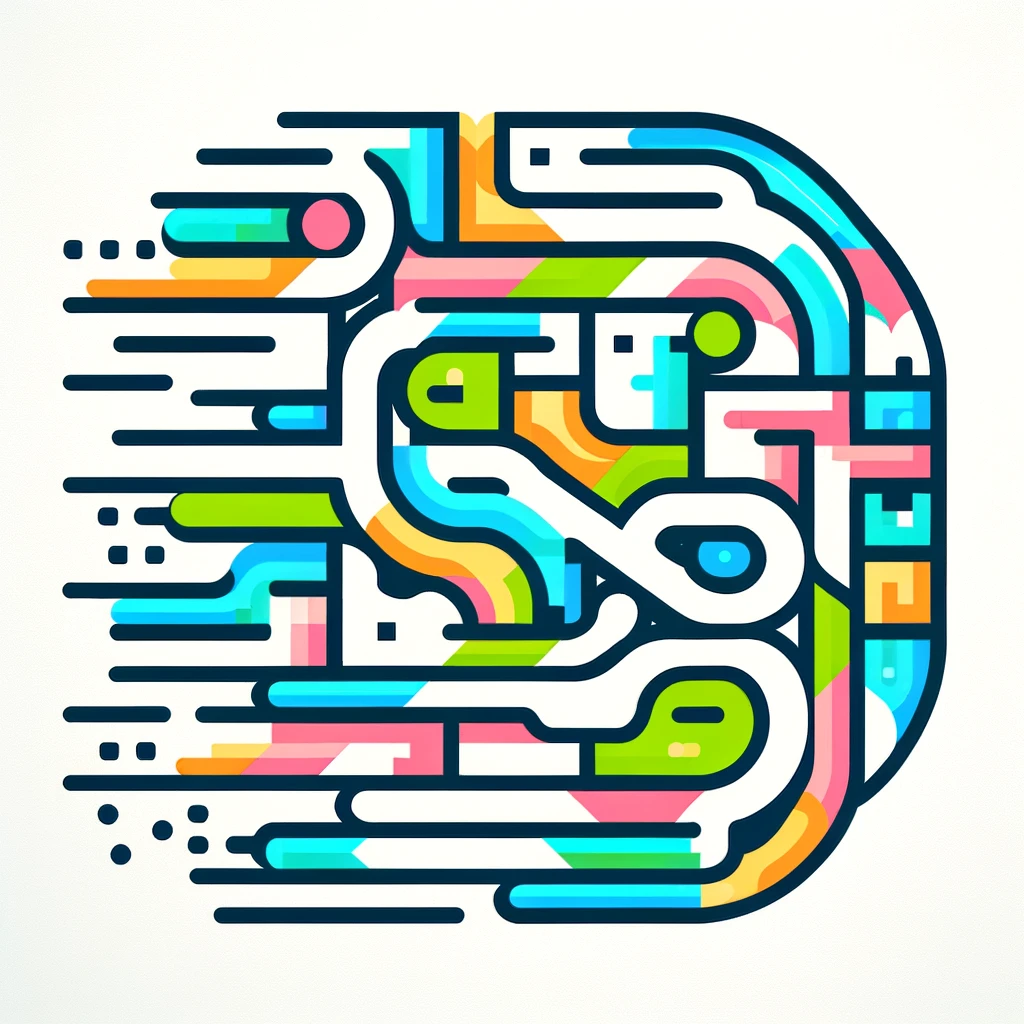
SHAP (SHapley Additive exPlanations)
SHAP (SHapley Additive exPlanations) is a method used to clarify how a machine learning model makes its predictions. It works by assigning each feature (or piece of information) a value that represents its contribution to the final decision. Think of it like breaking down a team effort: SHAP measures how much each member (feature) influences the outcome, considering all possible team arrangements. This helps users understand which factors are driving predictions, providing transparency and trust in complex models, especially when making important decisions.