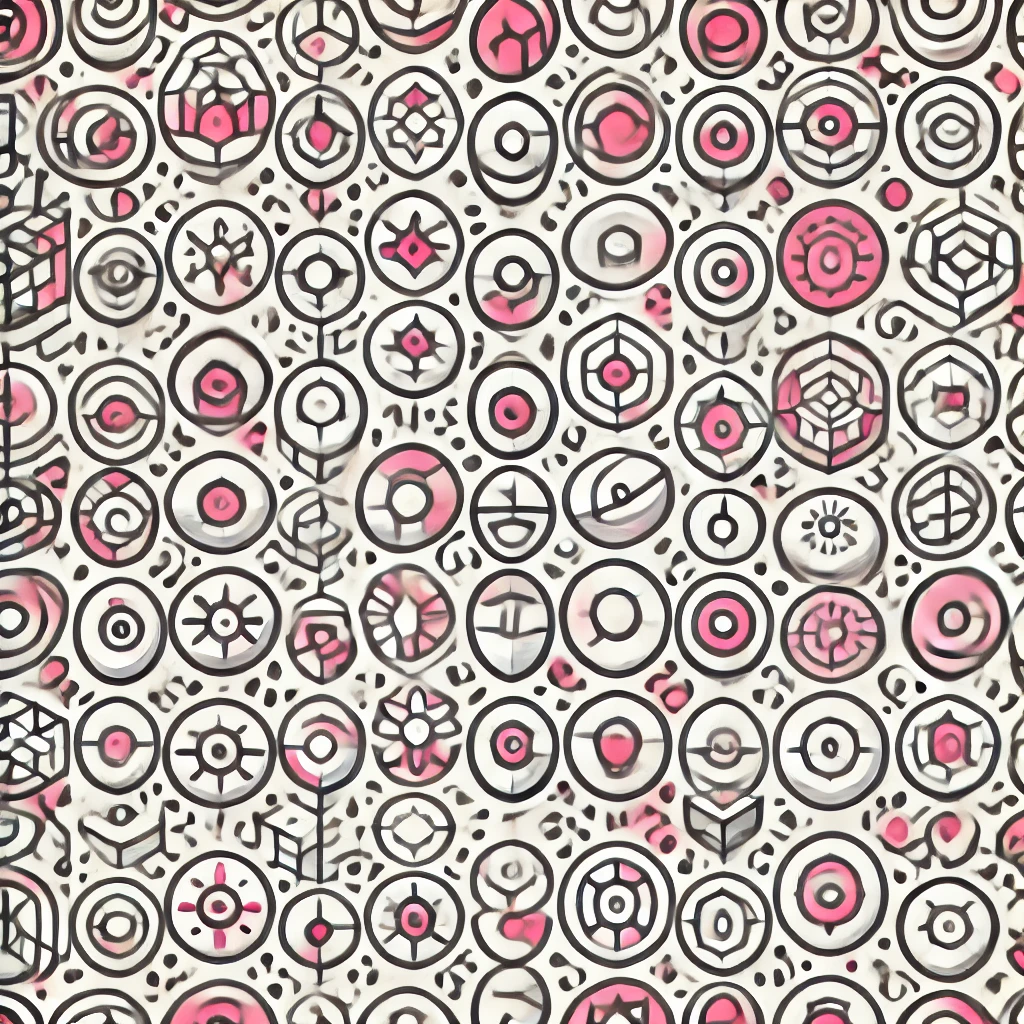
Semi-supervised Learning
Semi-supervised learning is a machine learning approach that uses a combination of a small amount of labeled data and a larger amount of unlabeled data to train models. Imagine having a few examples of something already identified and many unlabeled examples. The model learns from the labeled examples to understand patterns and then applies this knowledge to interpret the unlabeled data more efficiently. This method reduces the need for extensive manual labeling, saving time and resources, while still achieving effective performance, especially in situations where labeled data is scarce or expensive to obtain.