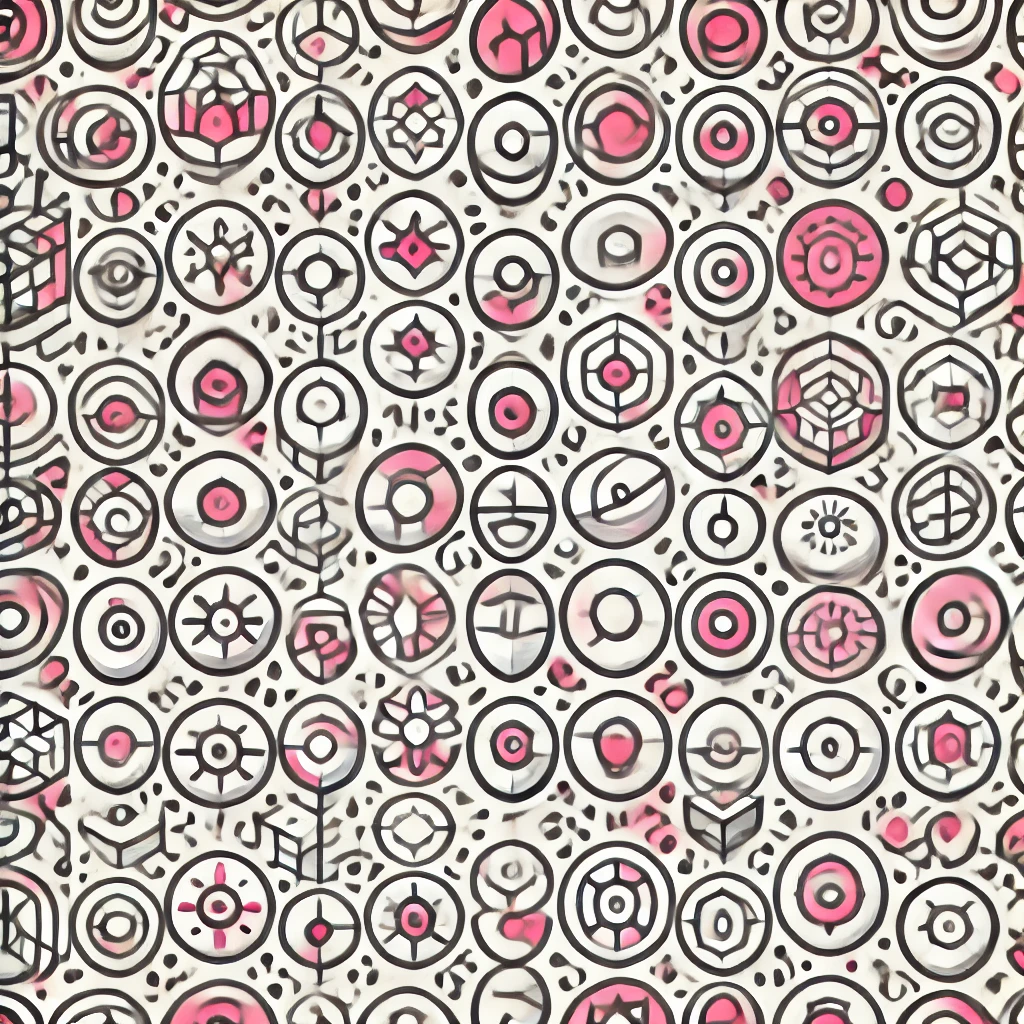
Permutation Importance
Permutation importance is a method used to determine how much each feature (or input variable) contributes to a predictive model's accuracy. It works by measuring the model's performance before and after randomly shuffling the values of a specific feature. If shuffling a feature significantly decreases the model’s accuracy, that feature is considered important. Conversely, if the performance remains largely unchanged, the feature has little impact. This approach helps identify which factors are most influential in making predictions, providing insights into the model's behavior and the relative importance of different variables.