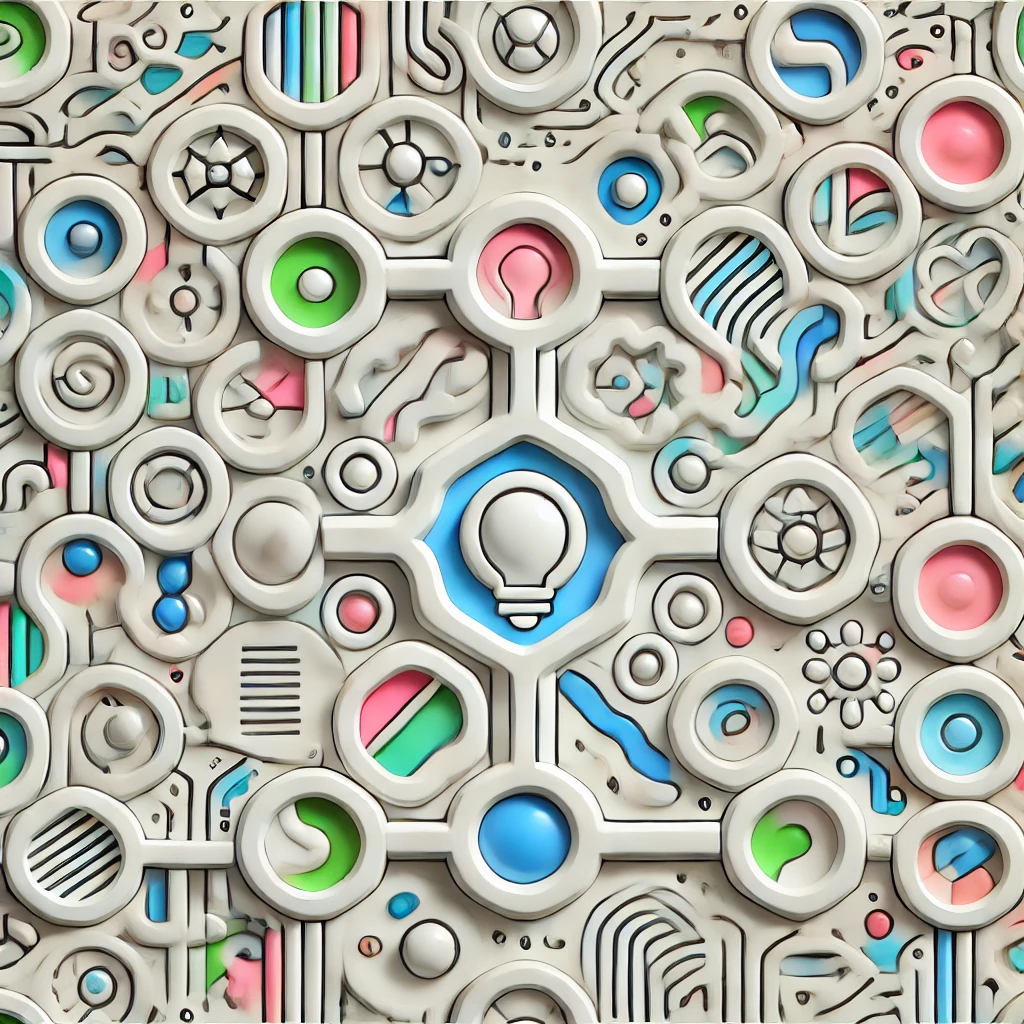
Penalized Likelihood
Penalized likelihood is a statistical method used to improve the accuracy of models by balancing fit and simplicity. It adds a penalty term to the usual likelihood (which measures how well a model explains the data), discouraging overly complex models that might fit the data too closely but don’t generalize well. This helps prevent overfitting by favoring simpler, more generalizable models, especially when dealing with many variables or limited data. Essentially, penalized likelihood guides the model to find the best explanation that is both accurate and parsimonious.