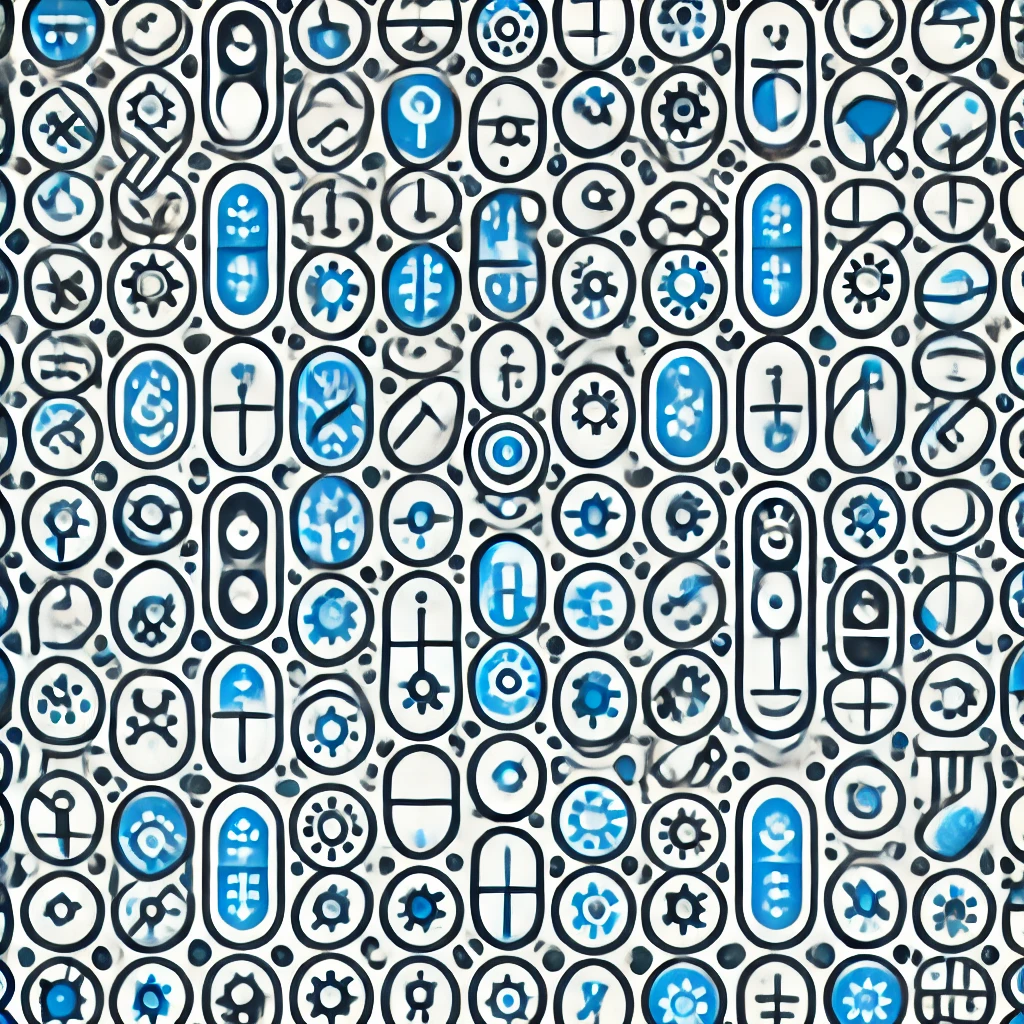
Off-Policy Learning
Off-policy learning is a method in machine learning where a system learns about or improves its decision-making policy using data collected from a different policy or way of acting. Instead of learning only from its own actions, it analyzes past experiences or external sources to evaluate and update its strategies. This approach allows the system to learn more efficiently, leverage existing data, and adapt without needing to perform all actions itself. It’s useful in scenarios where exploring new actions might be costly or risky, enabling safe learning from past or external information.