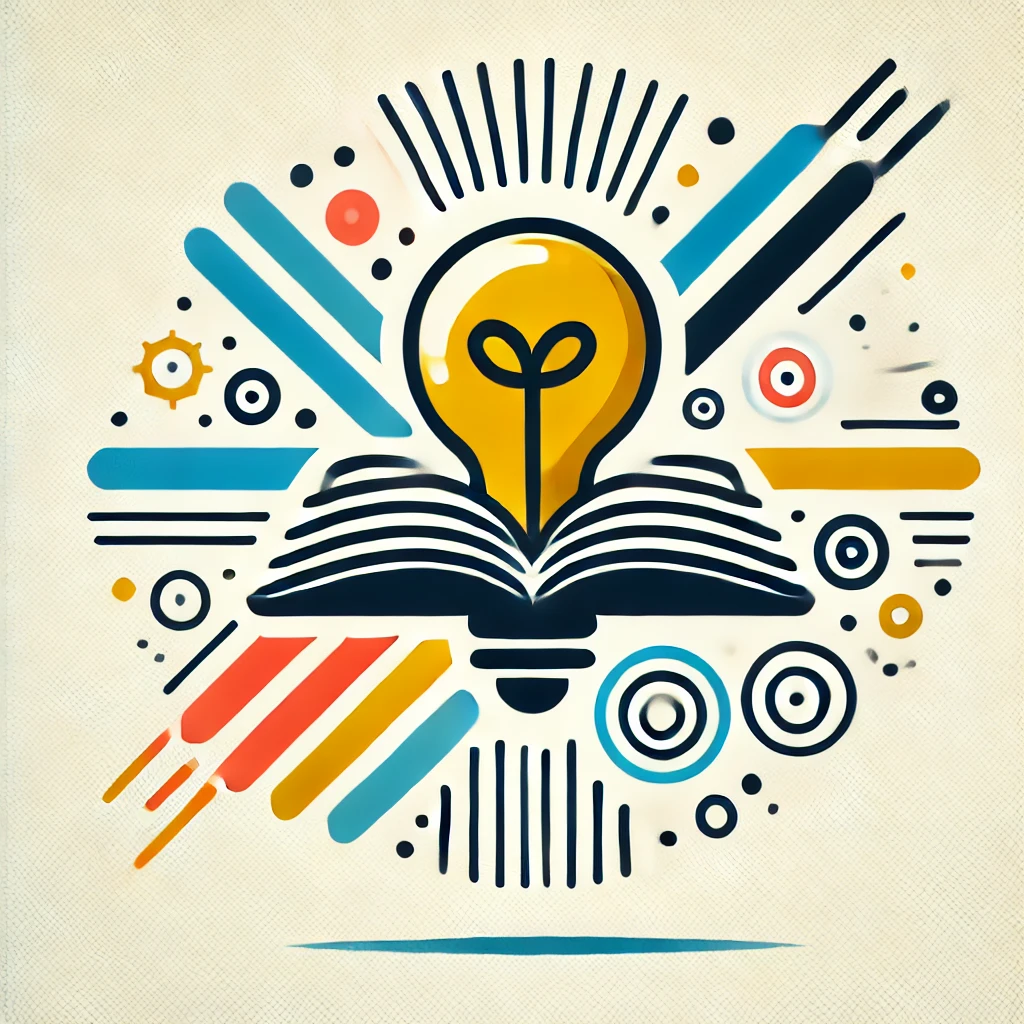
Model order selection
Model order selection is the process of choosing the number of parameters or components in a statistical or mathematical model to best represent data without overfitting or underfitting. Essentially, it's about finding the right complexity—enough to capture important patterns but not so much that the model becomes overly tailored to the specific dataset. Techniques like the Akaike Information Criterion (AIC) or Bayesian Information Criterion (BIC) help compare models with different orders and identify the most appropriate one, ensuring accurate predictions and reliable insights.