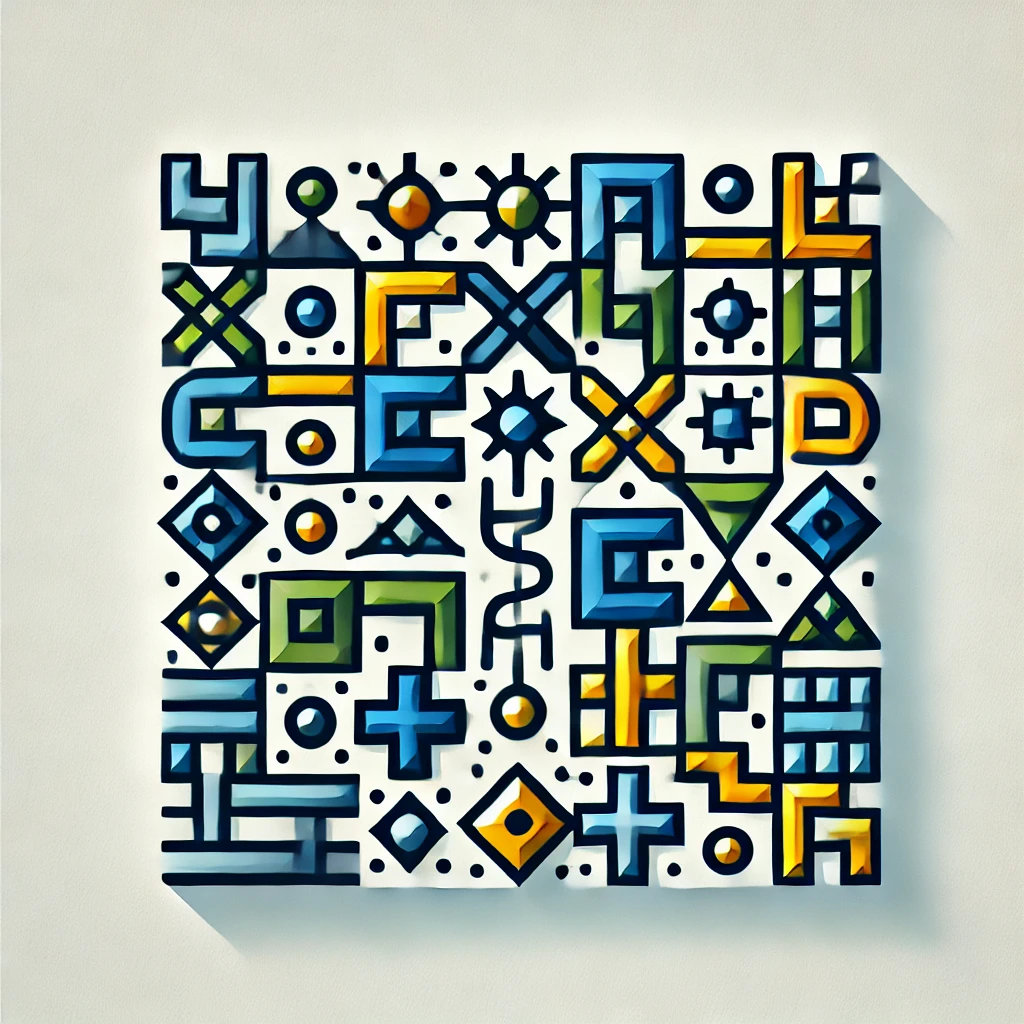
Model Interpretability
Model interpretability refers to how easily a person can understand and trust the decisions made by a machine learning model. It means being able to see which factors or data points influenced the model’s outcome, like understanding why a loan was approved or denied. Interpretable models help users and stakeholders gain confidence, identify potential biases, and ensure the model's reasoning aligns with real-world expectations. In essence, interpretability makes complex algorithms transparent and understandable, bridging the gap between technical processes and human comprehension.