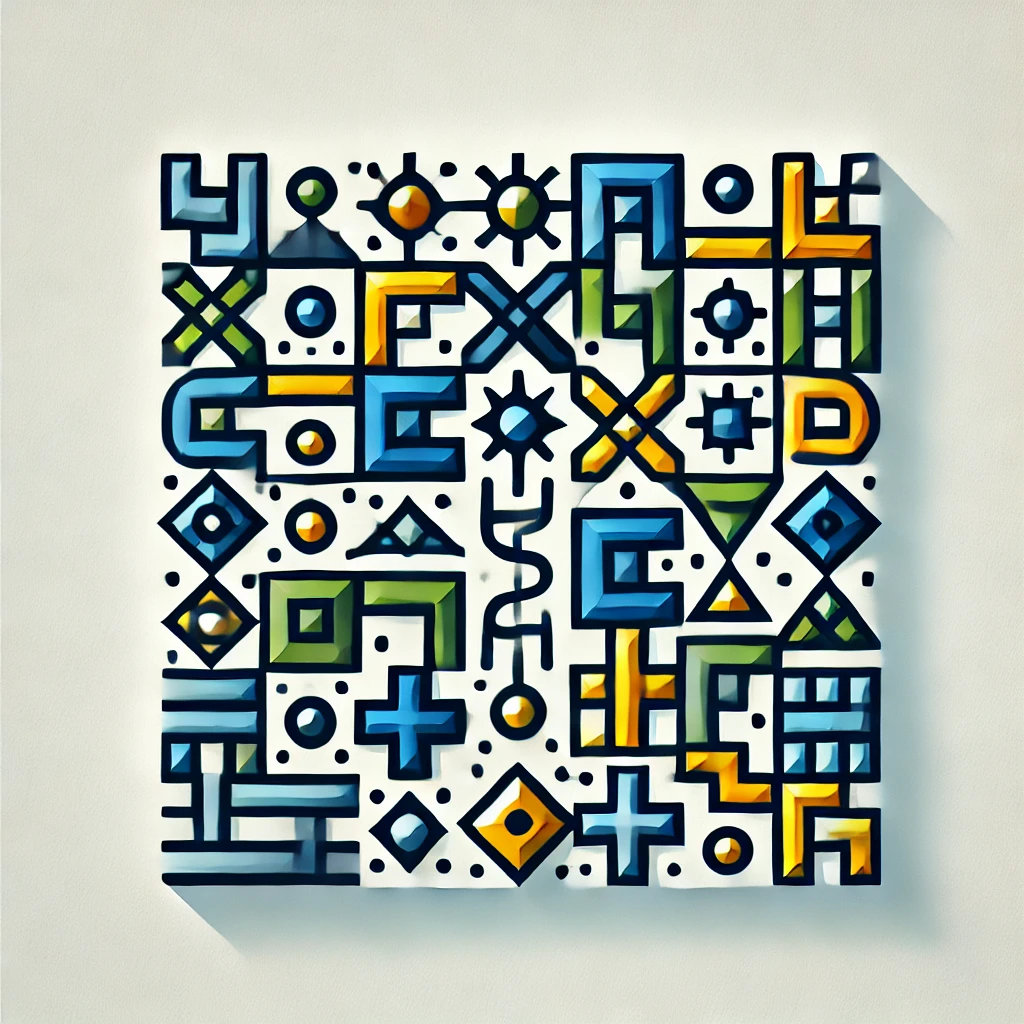
Model Diagnostics
Model diagnostics involve checking how well a statistical or machine learning model fits and performs on data. It helps identify if the model's assumptions are met, if there are any errors or biases, and whether it predicts accurately. Techniques include analyzing residuals (differences between predicted and actual values), checking for patterns indicating problems, and evaluating metrics like accuracy or error rates. Proper diagnostics ensure the model's results are reliable and valid, guiding improvements and confidence in decision-making based on the model's outputs.