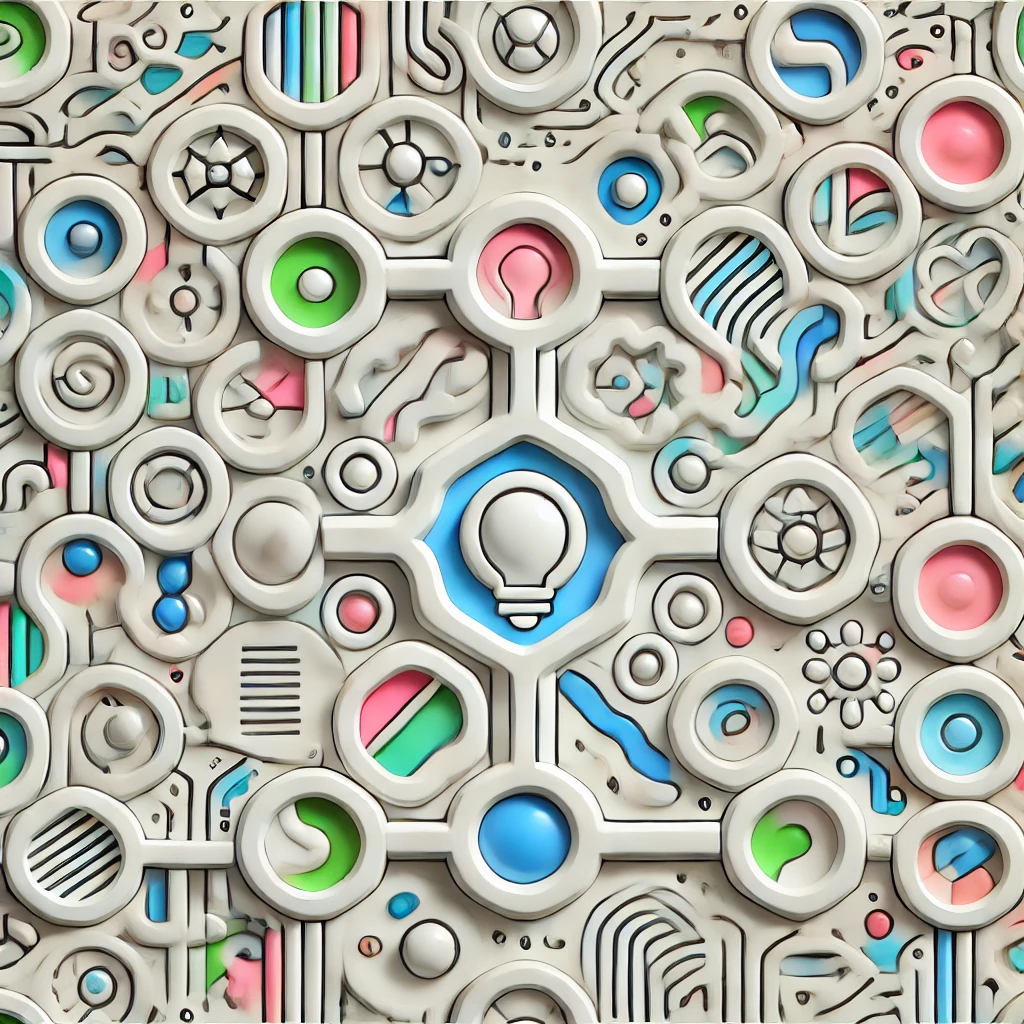
Machine Learning in Spectroscopy
Machine learning in spectroscopy involves using computer algorithms to analyze complex spectral data—like light or other signals—to identify patterns and make predictions. By training on known examples, these algorithms learn to classify materials, detect subtle differences, or quantify components accurately. This approach enhances traditional analysis by handling large, intricate datasets efficiently and revealing insights that might be difficult for humans to discern manually. Overall, machine learning automates and improves the interpretation of spectral information, leading to faster, more precise assessments across fields like chemistry, medicine, and environmental monitoring.