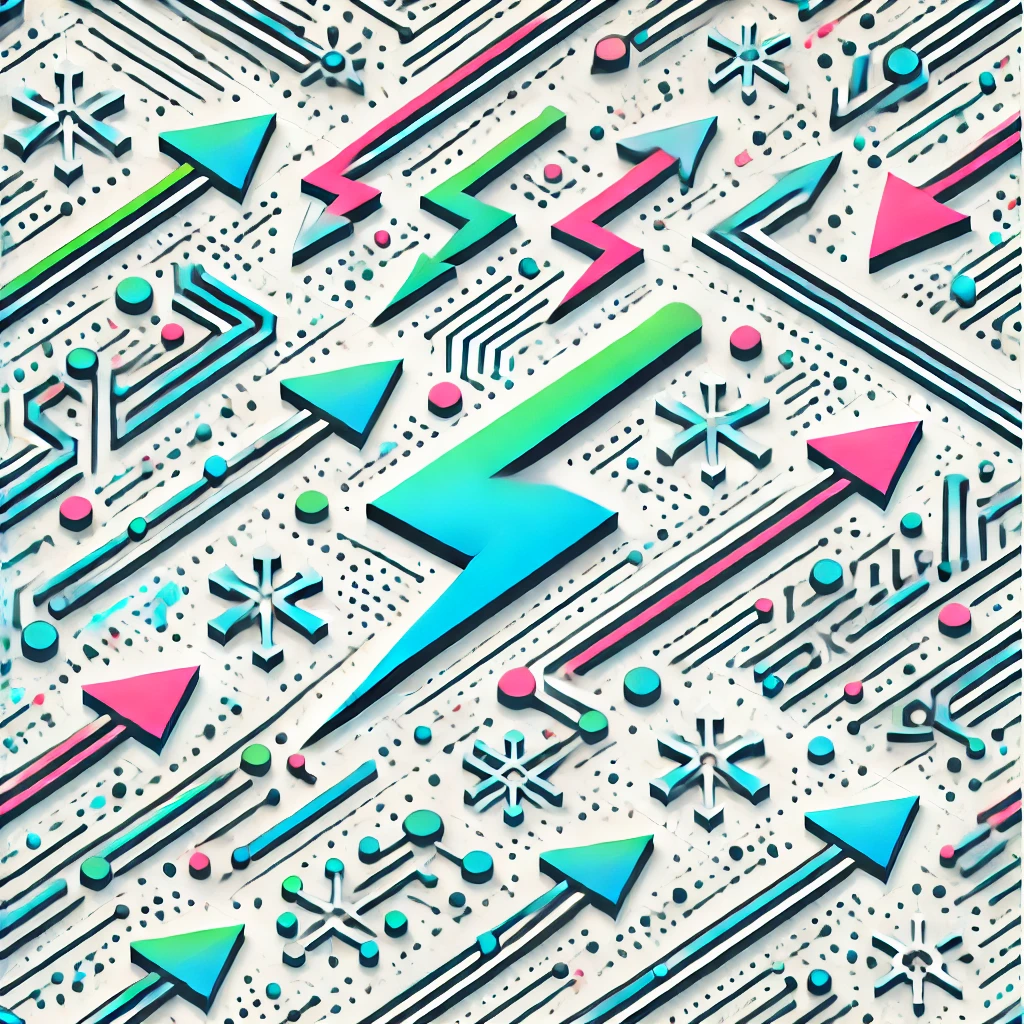
Lyapunov's central limit theorem
Lyapunov's Central Limit Theorem states that when you average a large number of independent random variables—each with its own average and spread—the overall average tends to follow a normal (bell-shaped) distribution, even if the original variables are not normally distributed. The theorem provides specific conditions related to the variables' variances and higher moments, ensuring the approximation improves as the number of variables increases. This principle explains why many real-world phenomena, like measurement errors or test scores, tend to form a normal distribution when multiple independent factors are combined.