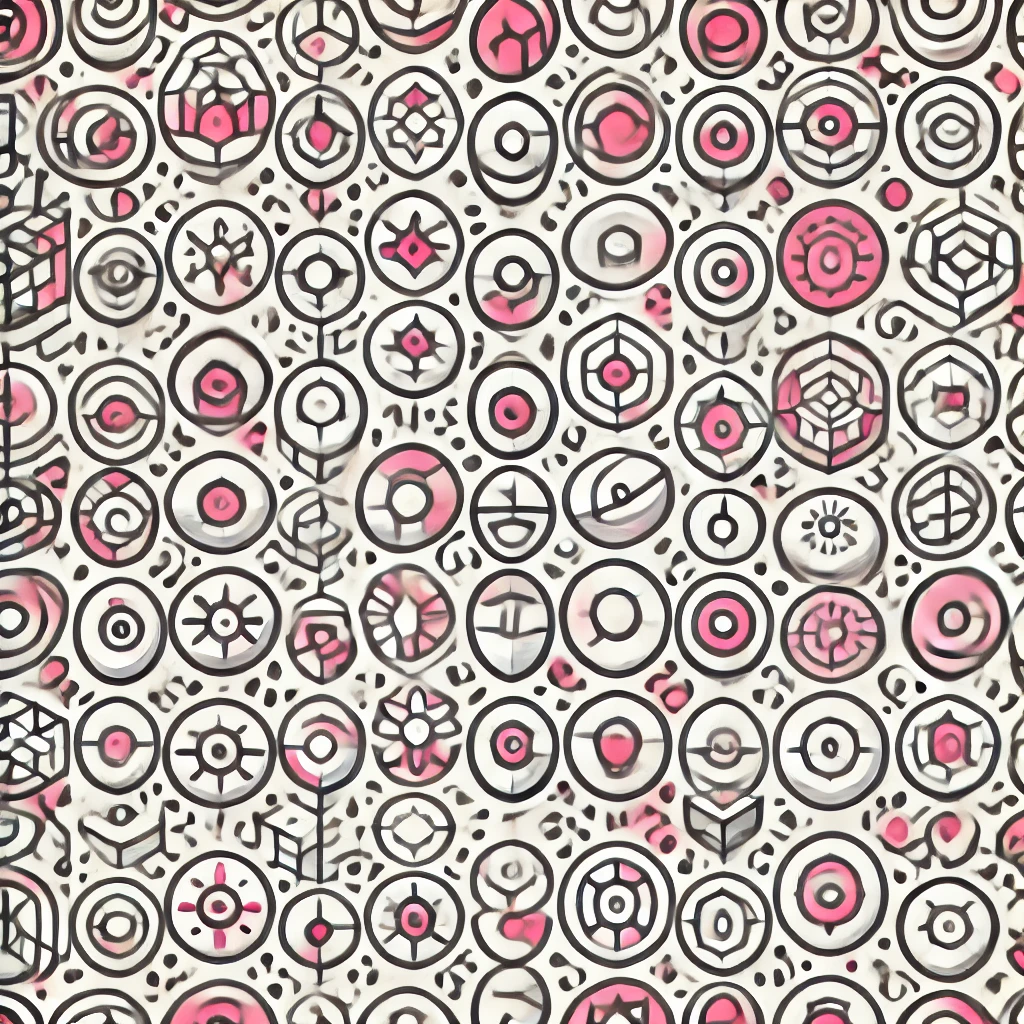
Kullback-Leibler Divergence
Kullback-Leibler (KL) divergence measures how one probability distribution differs from a second, expected distribution. Think of it as quantifying the information lost when you approximate the true data distribution with a simpler one. For example, if you have a model predicting weather patterns and the actual weather data, KL divergence tells you how much the model's predictions deviate from reality. It’s always non-negative and zero only when both distributions are identical. Essentially, KL divergence helps assess how close or far a model's assumptions are from the true underlying data.