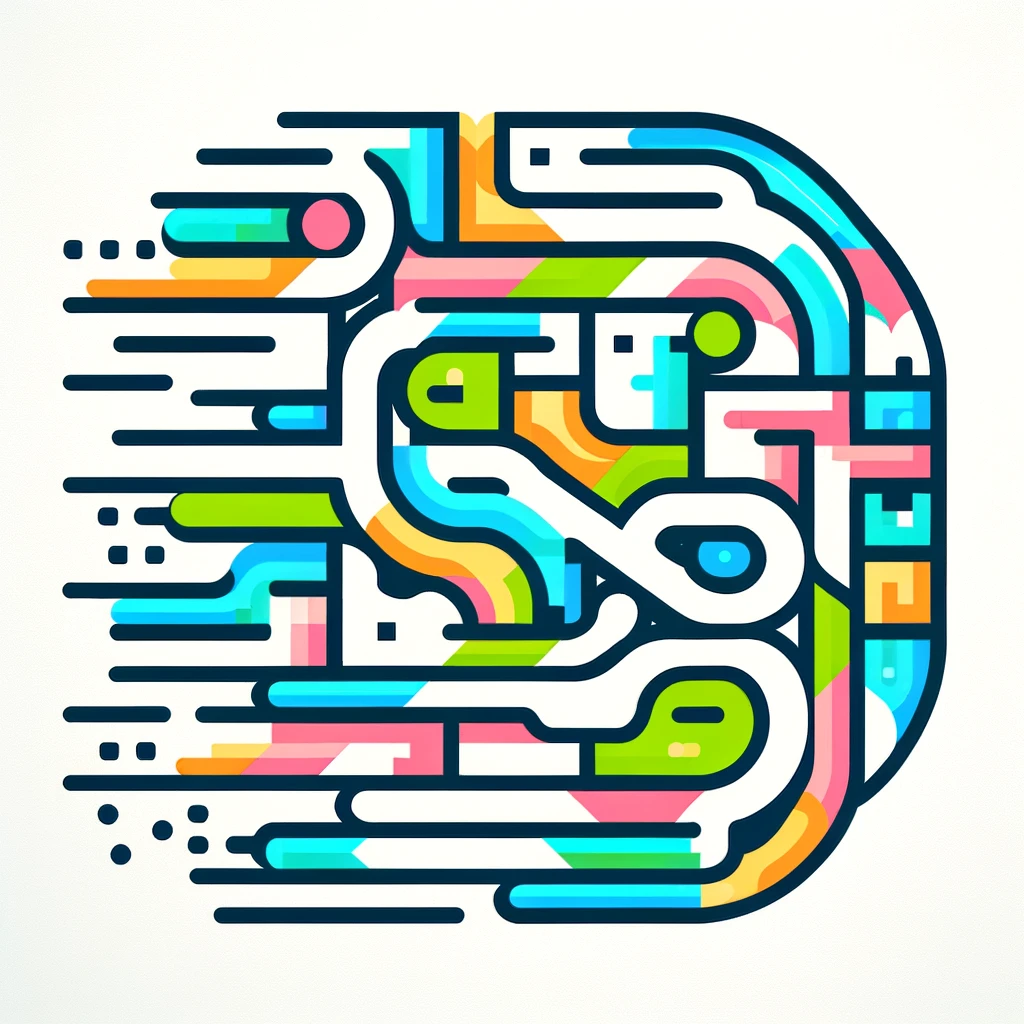
Kenyon and Knott
Kenyon and Knott developed a method called the "kernel" function in support vector machines (SVMs), which allows algorithms to efficiently classify data that isn't linearly separable. Essentially, they mapped complex data into higher-dimensional spaces where a clear dividing boundary exists, making it easier to distinguish categories. Their work enables SVMs to handle complicated patterns by implicitly calculating these transformations, improving accuracy without excessive computational costs. This approach is fundamental in machine learning for tasks like image recognition and bioinformatics, where data often exhibits intricate relationships.