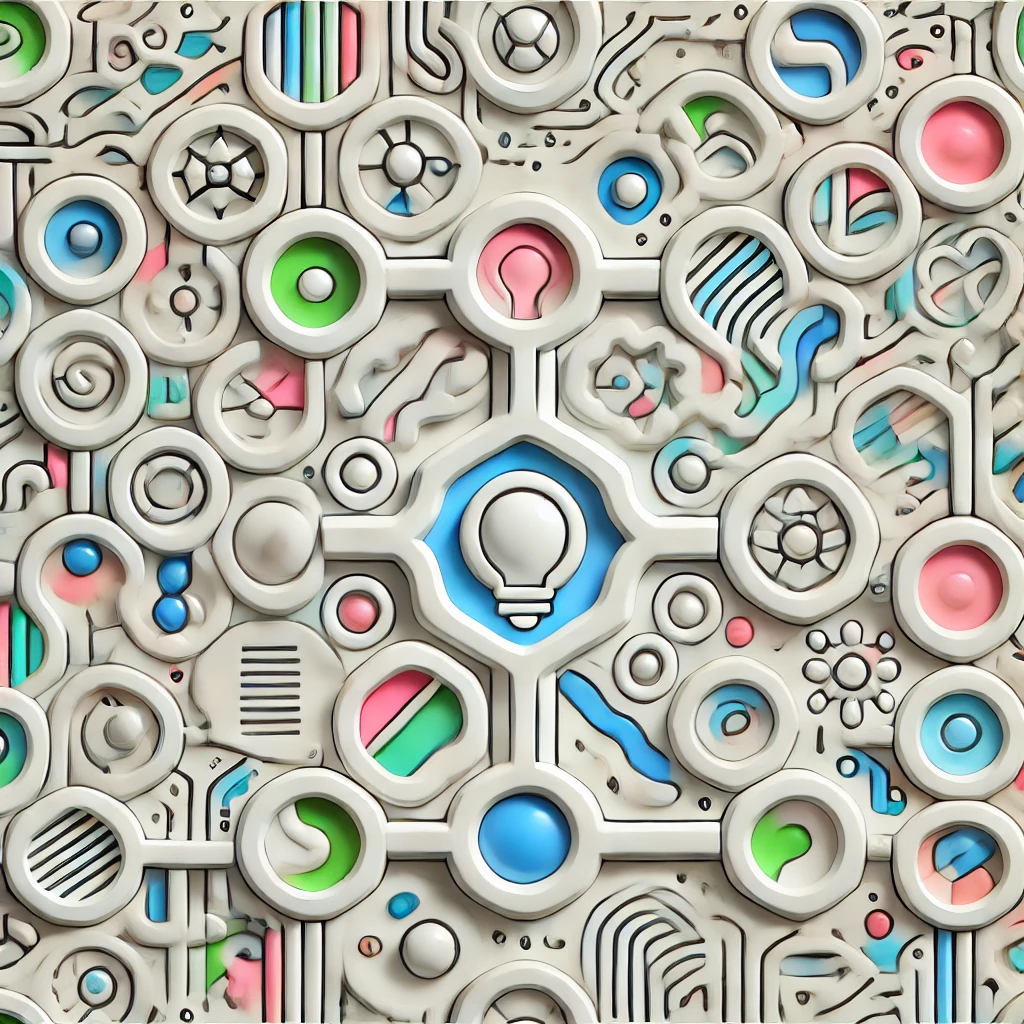
Kalman gain
Kalman gain is a factor used in data filtering and estimation that determines how much trust to place in new measurements versus previous predictions. When estimating a system’s state (like position or speed), the Kalman gain adjusts the influence of incoming data depending on its accuracy. If new measurements are reliable, the gain increases, giving them more weight. If the data is noisy or uncertain, the gain decreases, relying more on the prior prediction. Essentially, it optimally balances trusting current measurements against past estimates to produce the most accurate overall understanding of the system.