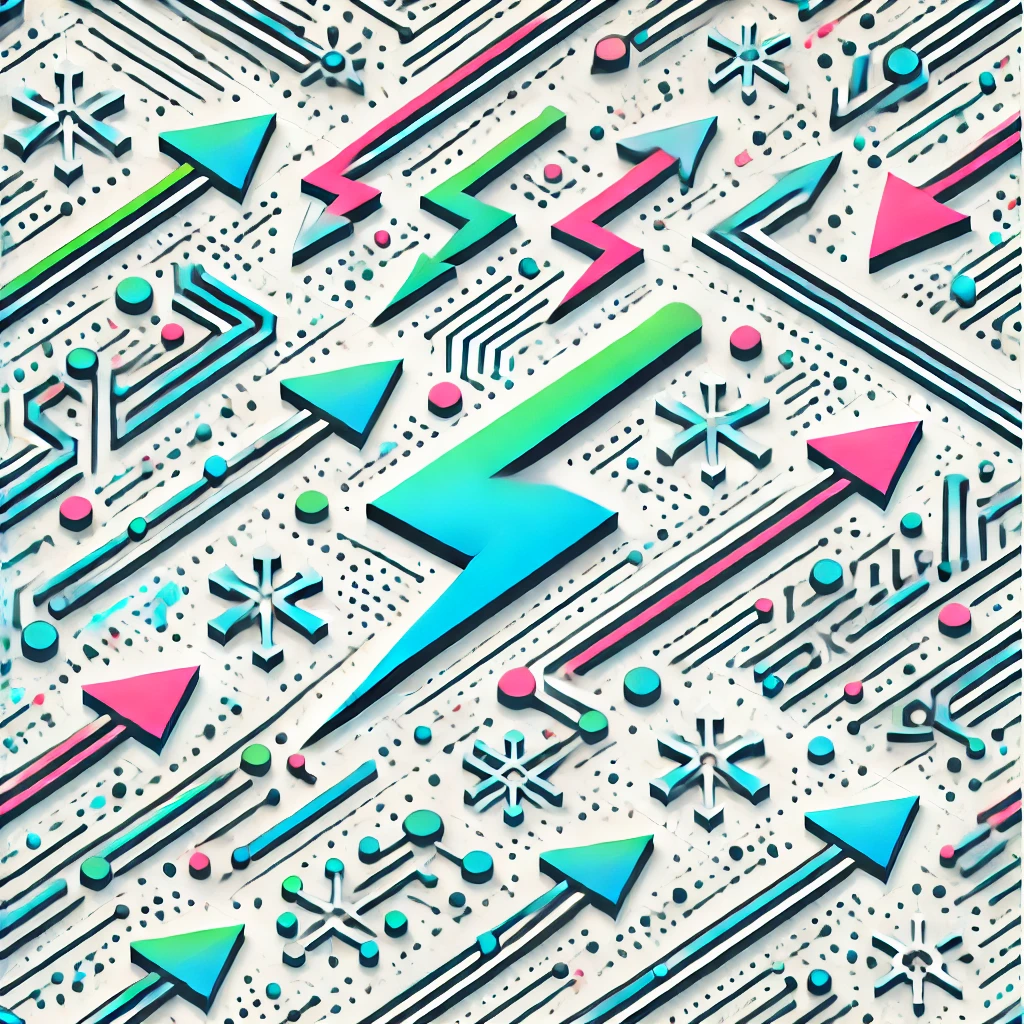
JSL Methodology
The JSL (Joint Sample and Label) methodology is a strategic approach for efficiently collecting data in machine learning. It involves selecting a smaller, representative subset of data (sampling) and accurately assigning labels (annotations) to this subset. This way, models can learn effectively without needing to process the entire dataset, saving time and resources. The key is to carefully choose the right samples and labels, ensuring the model gains comprehensive understanding while reducing effort and cost. Overall, JSL optimizes data acquisition for better machine learning performance in a cost-effective and scalable manner.