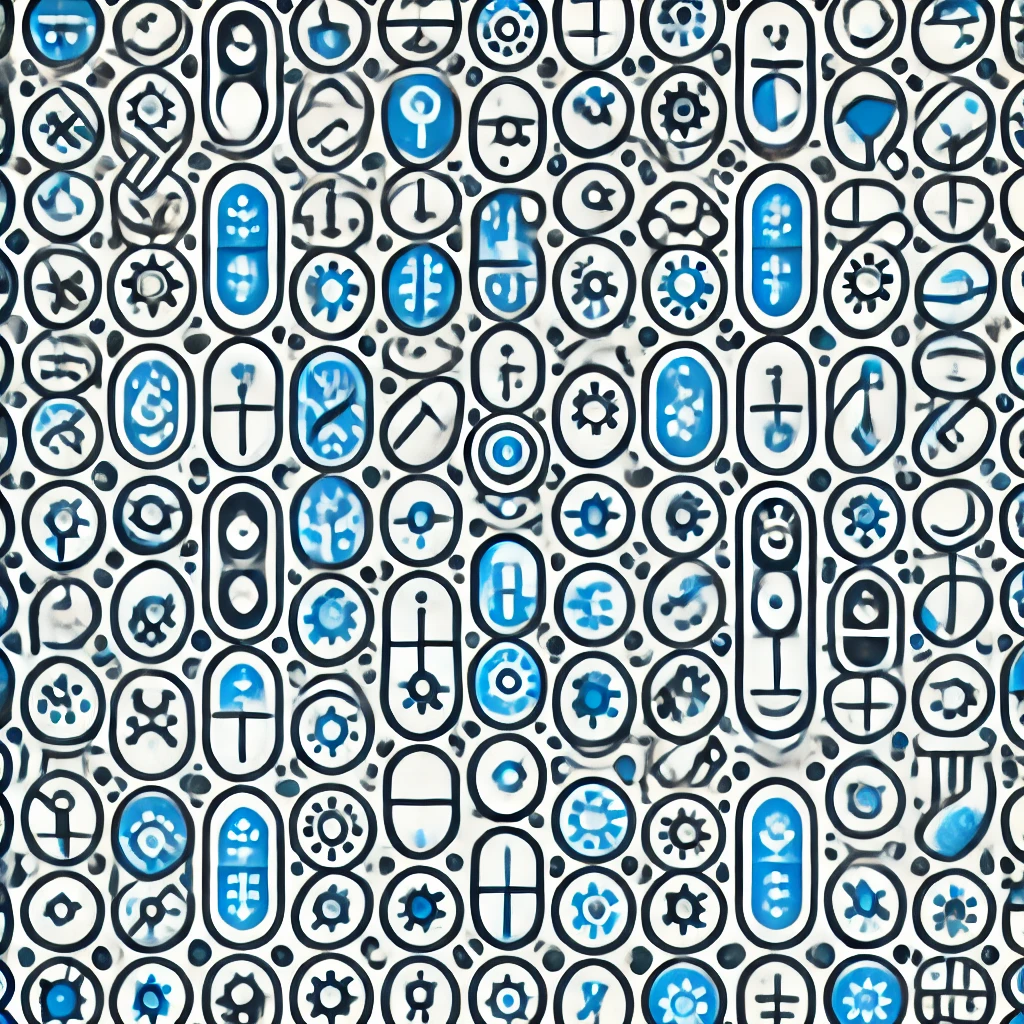
JMI
JMI, or Joint Mutual Information, is a technique used in feature selection to identify the most relevant variables for a machine learning model. It measures how much information multiple features share collectively with the target outcome. By considering the combined relevance of features together, JMI helps select a subset that provides the most predictive power without redundancy, improving model performance and interpretability. Essentially, it finds the best group of features that work well together to explain or predict the target variable efficiently.