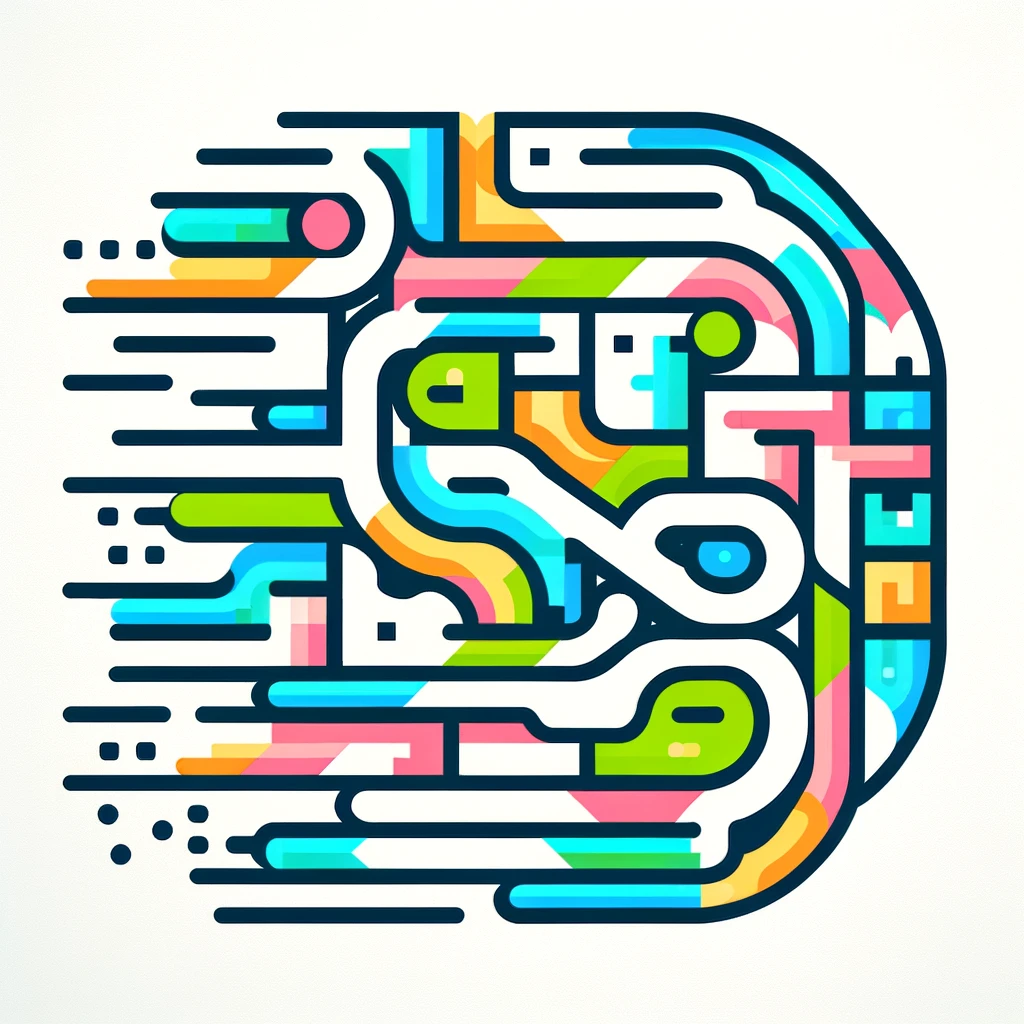
Jeffrey's prior
Jeffrey's prior, named after the statistician Harold Jeffreys, is a method for assigning probabilities when there is little prior information about a particular parameter. Unlike other priors that might be subjective, Jeffrey's prior is based on the likelihood of observing data. It uses the concept of "invariant" measures, meaning it remains consistent regardless of transformations of the data. This approach helps ensure that prior beliefs do not unduly influence the outcome, allowing for a more objective analysis, particularly useful in Bayesian statistics when integrating new evidence with existing knowledge.