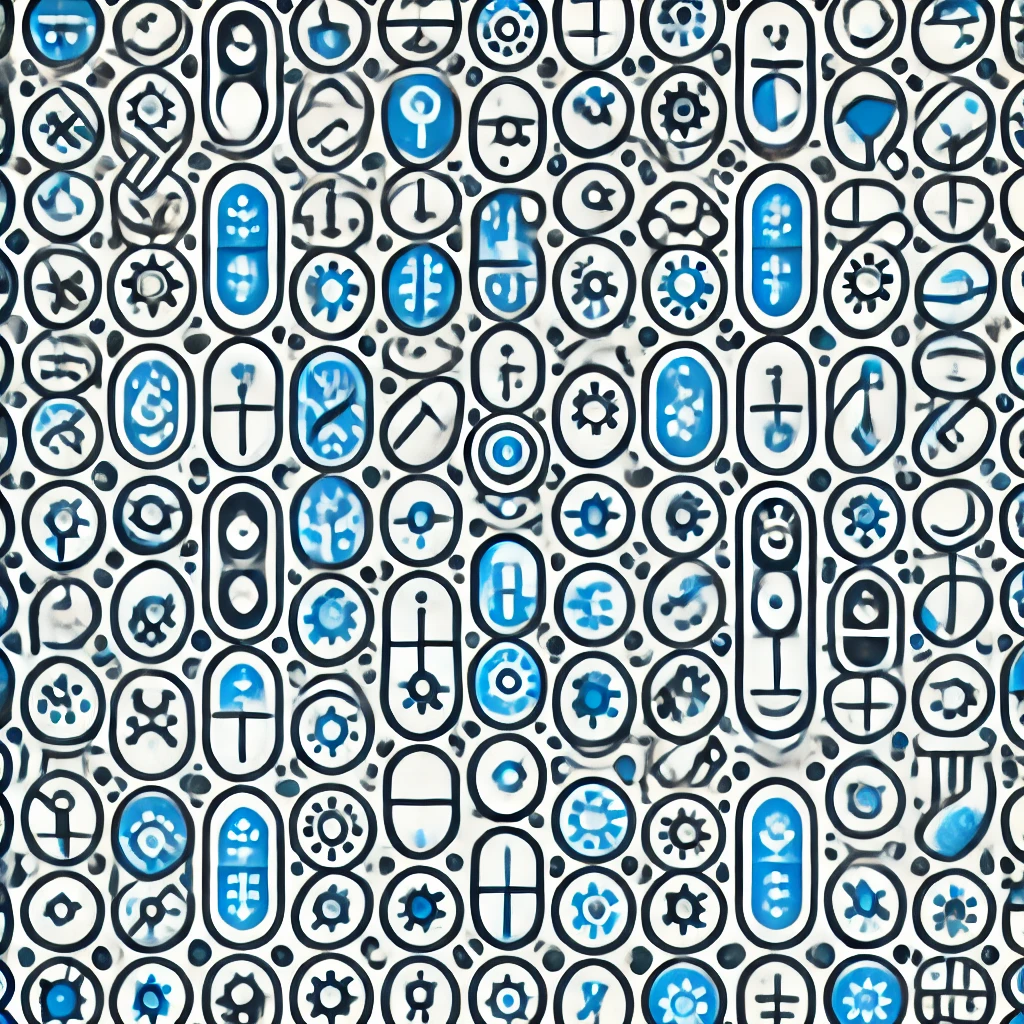
Huber's theory
Huber's theory in statistics addresses how to best estimate a central value (like a mean) when data includes outliers or errors. Traditional methods like the average can be heavily influenced by extreme values, skewing results. Huber proposed a robust approach that combines the sensitivity of the mean with the resilience of median, reducing the influence of outliers. It uses a function to limit the impact of very large deviations, providing a more reliable estimate of the central tendency in contaminated or imperfect data. This approach is widely used in real-world data analysis where outliers are common but should not disproportionately affect results.