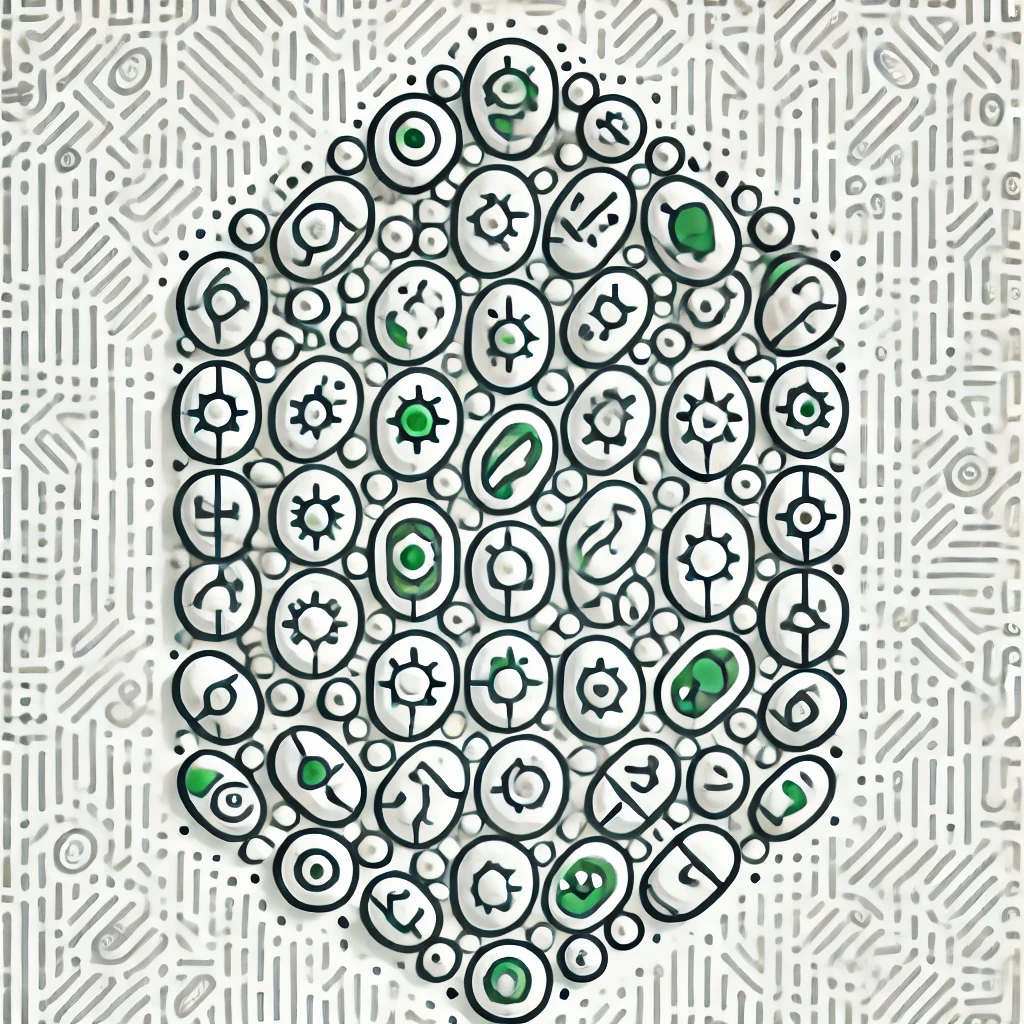
Hinge Loss
Hinge loss is a way to measure how well a classification model, like one sorting emails as spam or not, is performing. It focuses on ensuring that correct predictions are made with a comfortable margin of confidence. If the model makes a correct prediction with enough certainty, hinge loss is low or zero. If it’s wrong or barely confident, hinge loss increases, signaling the model needs improvement. This loss function encourages the model to not only be accurate but also confident, leading to more robust and reliable classifications.