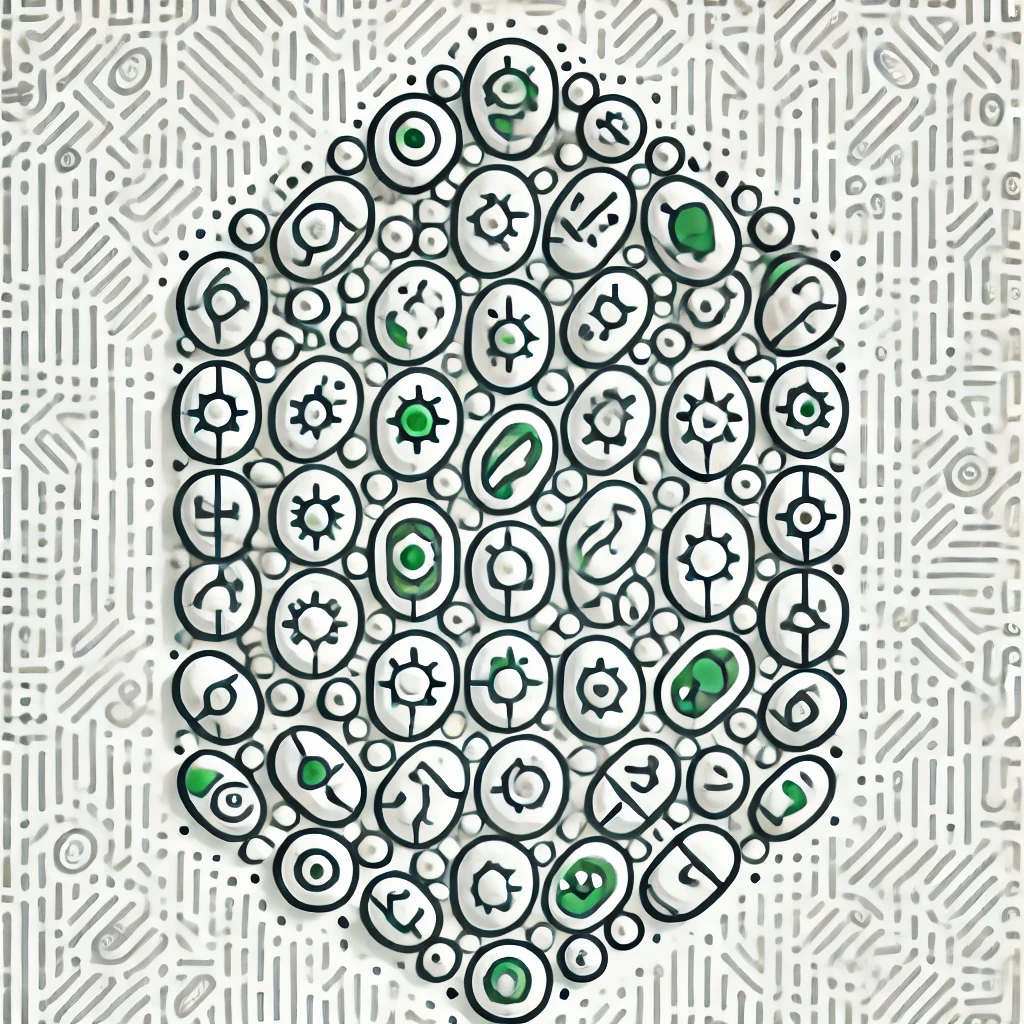
Generalization Error
Generalization error is the difference between a model’s predictions on new, unseen data and the true outcomes. It reflects how well a model learned the underlying patterns rather than just memorizing the training data. A low generalization error indicates the model can make accurate predictions on future data, while a high error suggests it may be overfitted or not capturing the true relationships. In essence, it measures a model’s ability to perform reliably outside of the data it was trained on, ensuring its usefulness in real-world applications.