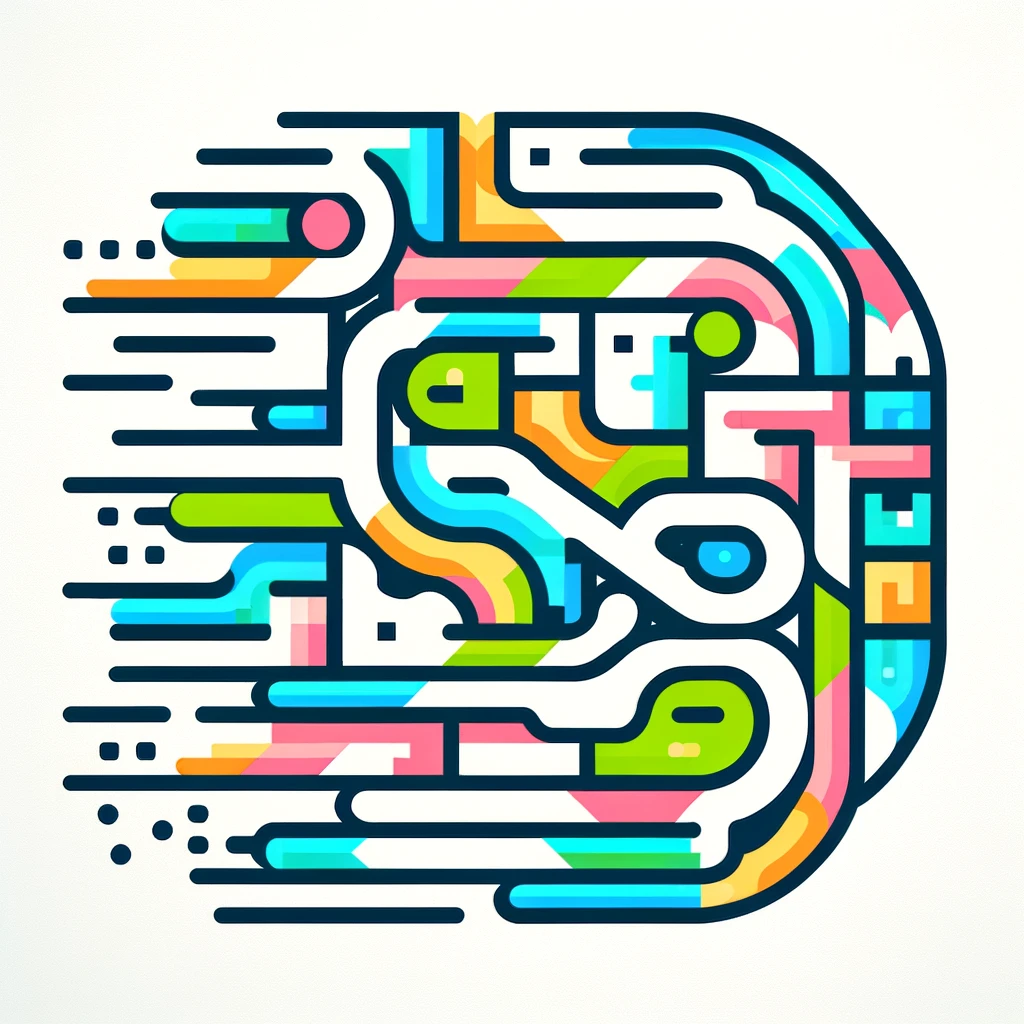
Expectation-Maximization (EM)
Expectation-Maximization (EM) is an iterative algorithm used to find the best estimates for models with hidden or unknown data. It works in two steps: the "Expectation" step estimates the missing information based on current parameters, and the "Maximization" step updates these parameters to best fit the observed data. Repeating these steps gradually improves the model's accuracy. EM is commonly used in situations like clustering or density estimation, where some data aspects are unobserved, enabling better model learning despite incomplete information.