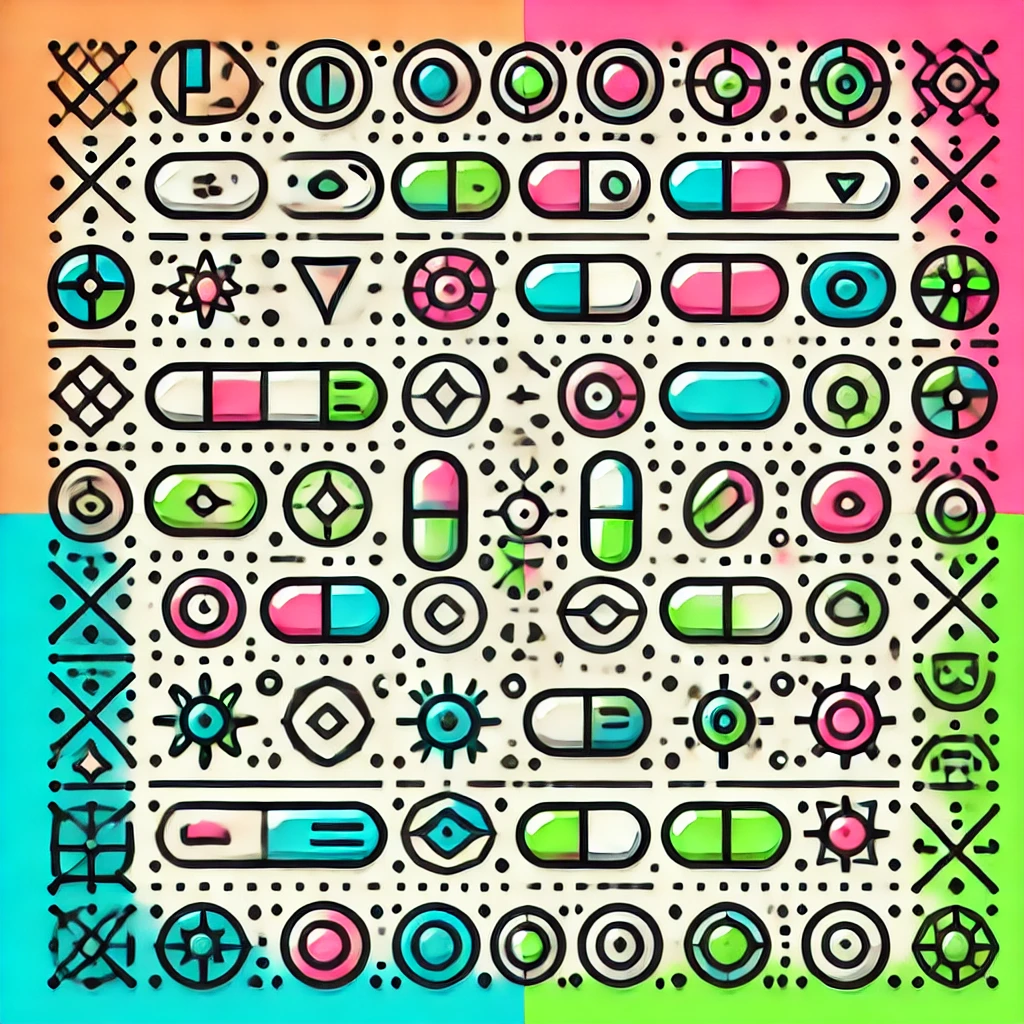
Dynamic Bayesian Networks
Dynamic Bayesian Networks (DBNs) are statistical models that represent how uncertain factors change over time. They extend traditional Bayesian networks by incorporating time, allowing us to track evolving relationships among variables—like weather, stock prices, or health metrics. Think of them as a series of connected snapshots, where each snapshot captures the state at a specific moment, and links between snapshots show how past events influence future states. This structure helps in predicting future outcomes, understanding complex systems, and making decisions based on sequences of data in fields such as engineering, finance, and medicine.