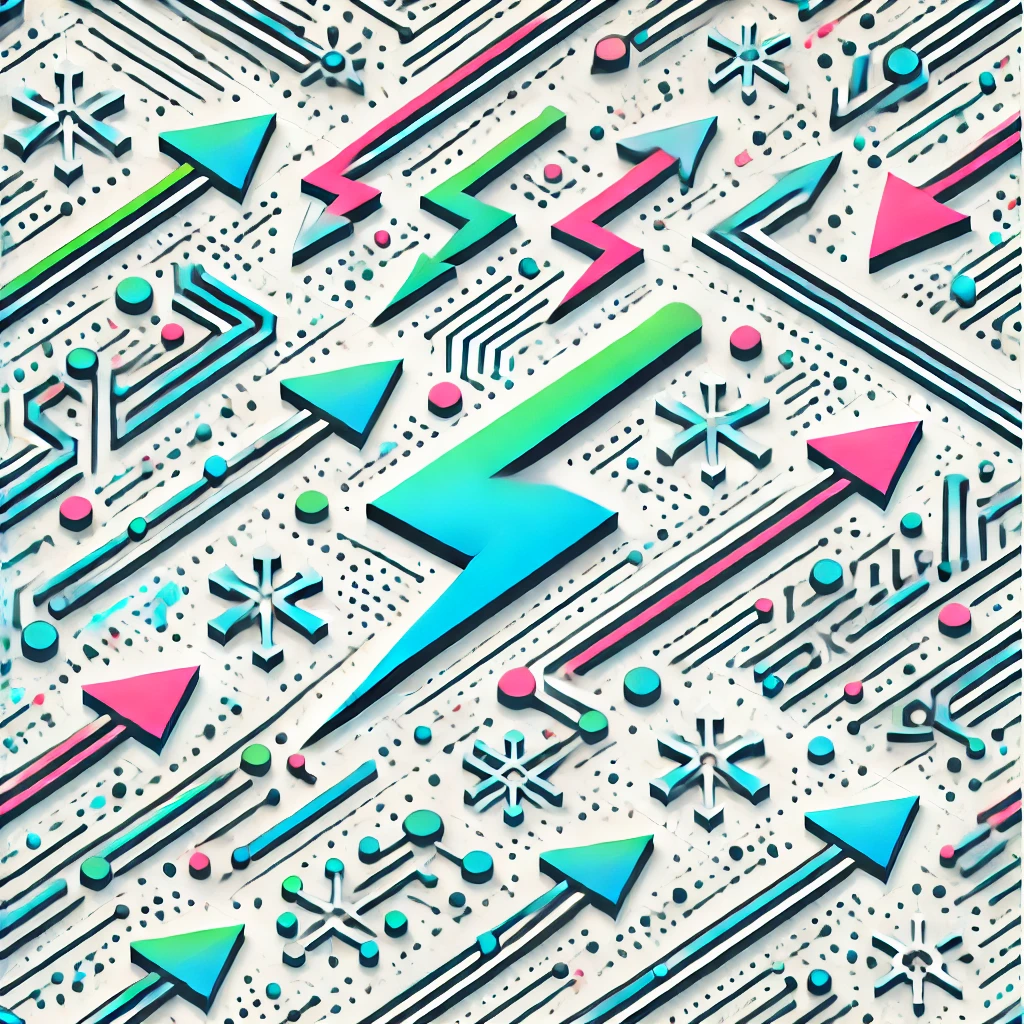
Dimensionality Reduction
Dimensionality reduction is a process used in data analysis that simplifies complex data by reducing the number of variables or features while retaining important information. Imagine trying to understand a large, intricate map; by focusing only on key routes and landmarks, you make navigation easier. In similar fashion, dimensionality reduction helps highlight the most significant patterns in vast datasets, making analysis more efficient and insightful. Techniques like Principal Component Analysis (PCA) or t-SNE are commonly used to achieve this, helping researchers and analysts visualize data better and improve performance in tasks like machine learning.