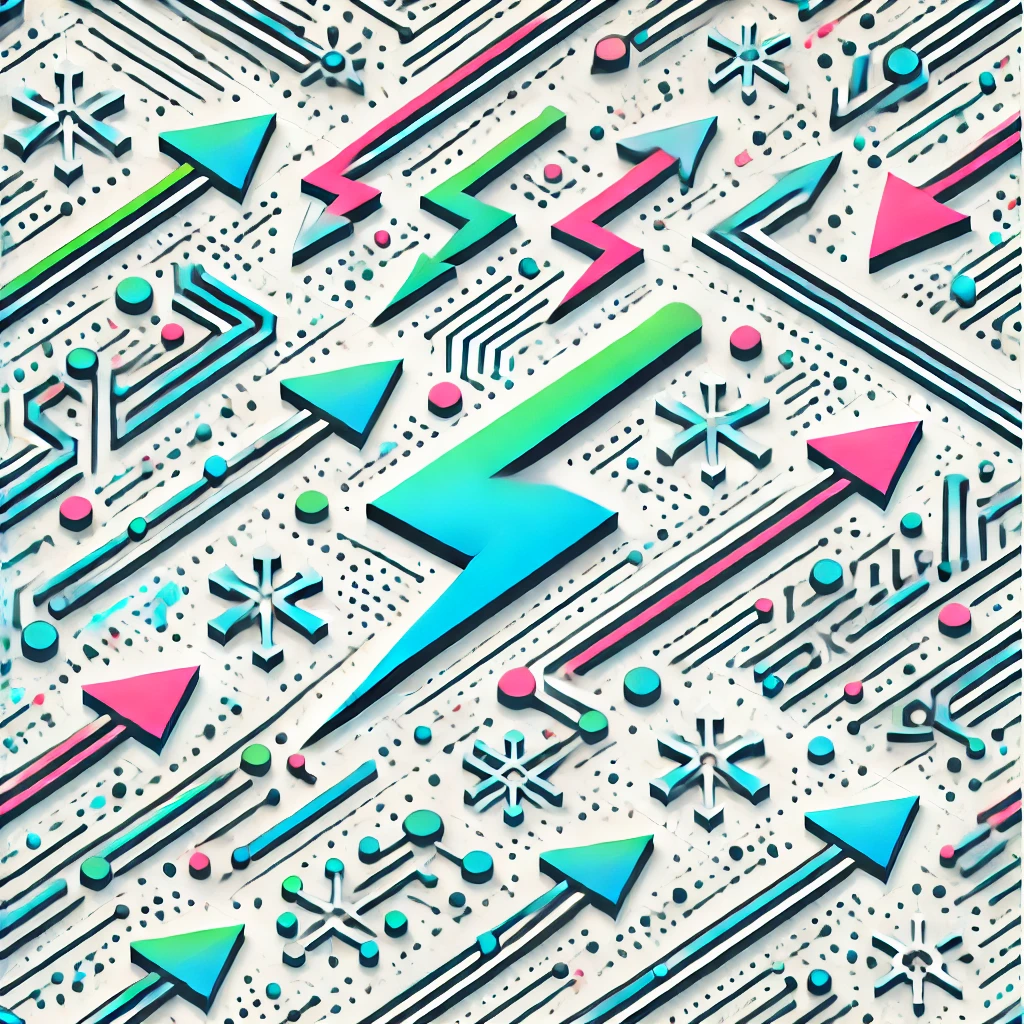
Defensive Distillation
Defensive distillation is a technique used to make machine learning models, especially neural networks, more resistant to attacks or manipulations. It involves training the model in two steps: first, teaching it to recognize patterns in data, and then using its outputs to create a simpler, smoother version of the model. This second model is less sensitive to small, malicious changes that might trick it. Essentially, defensive distillation makes the AI more robust and reliable by reducing its vulnerability to attempts at misleading or deceiving it.