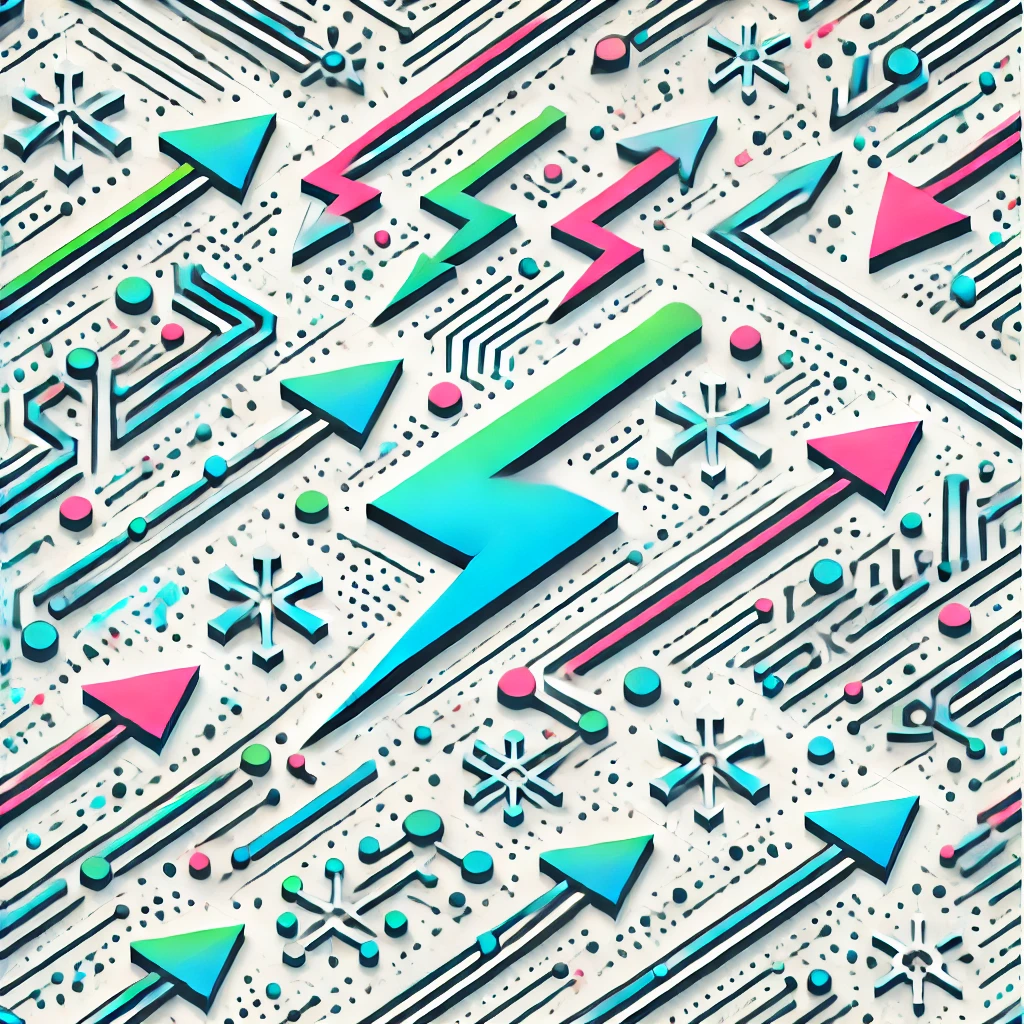
Cross-validation in clustering
Cross-validation in clustering is a method used to assess how well a clustering model will perform on new, unseen data. It involves dividing the dataset into several parts, training the clustering algorithm on some parts, and then testing how well it groups data in the remaining parts. This process helps determine if the clusters are stable and meaningful, rather than just fitting noise or specific data quirks. Essentially, it’s a way to validate the reliability of the clusters found, ensuring they reflect genuine patterns in the data.