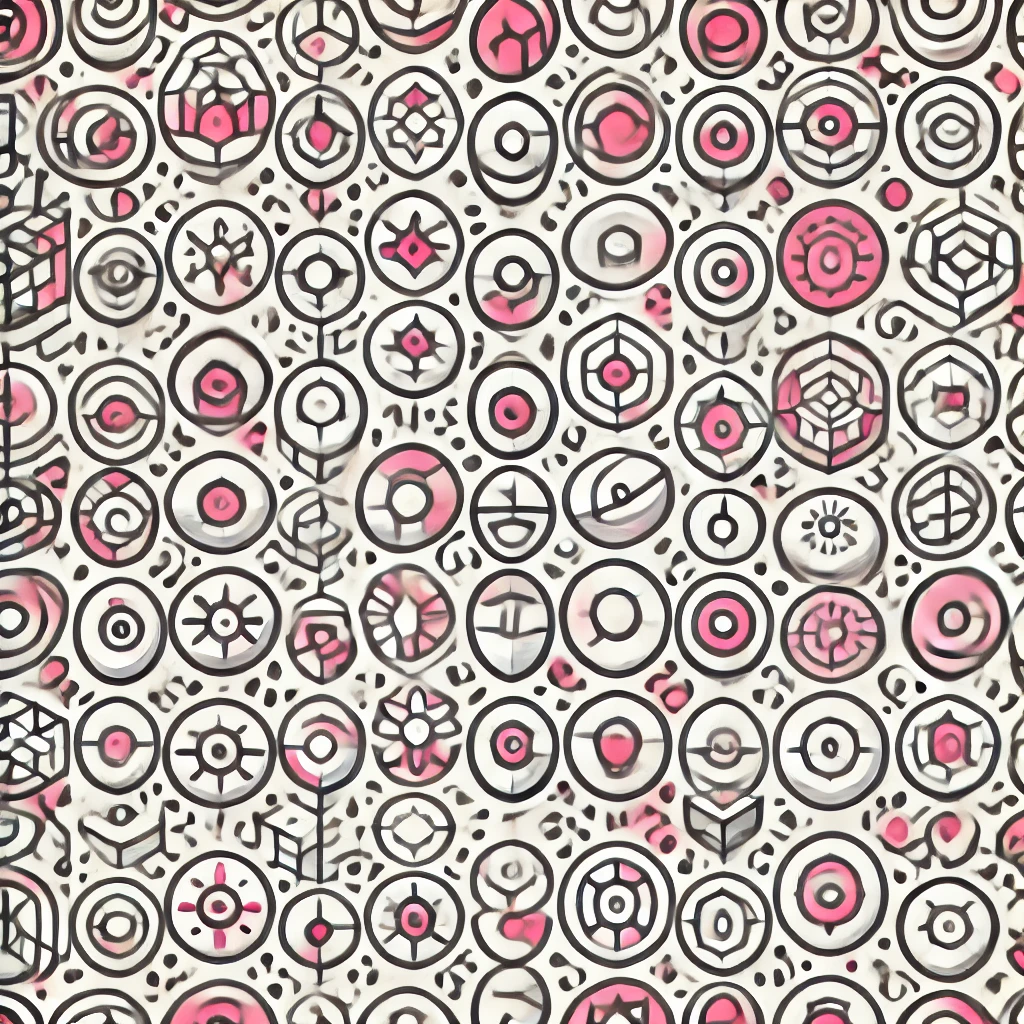
Classification performance
Classification performance measures how well a model correctly identifies different categories or groups within data. It involves evaluating metrics such as accuracy (how many predictions were correct), precision (how many positive predictions were true), recall (how many actual positives were correctly identified), and F1-score (a balance between precision and recall). These metrics help determine the reliability and effectiveness of the model in sorting data into the right classes, ensuring it performs well in real-world applications like spam detection, medical diagnosis, or customer segmentation. Good classification performance means the model makes accurate, consistent, and useful predictions.