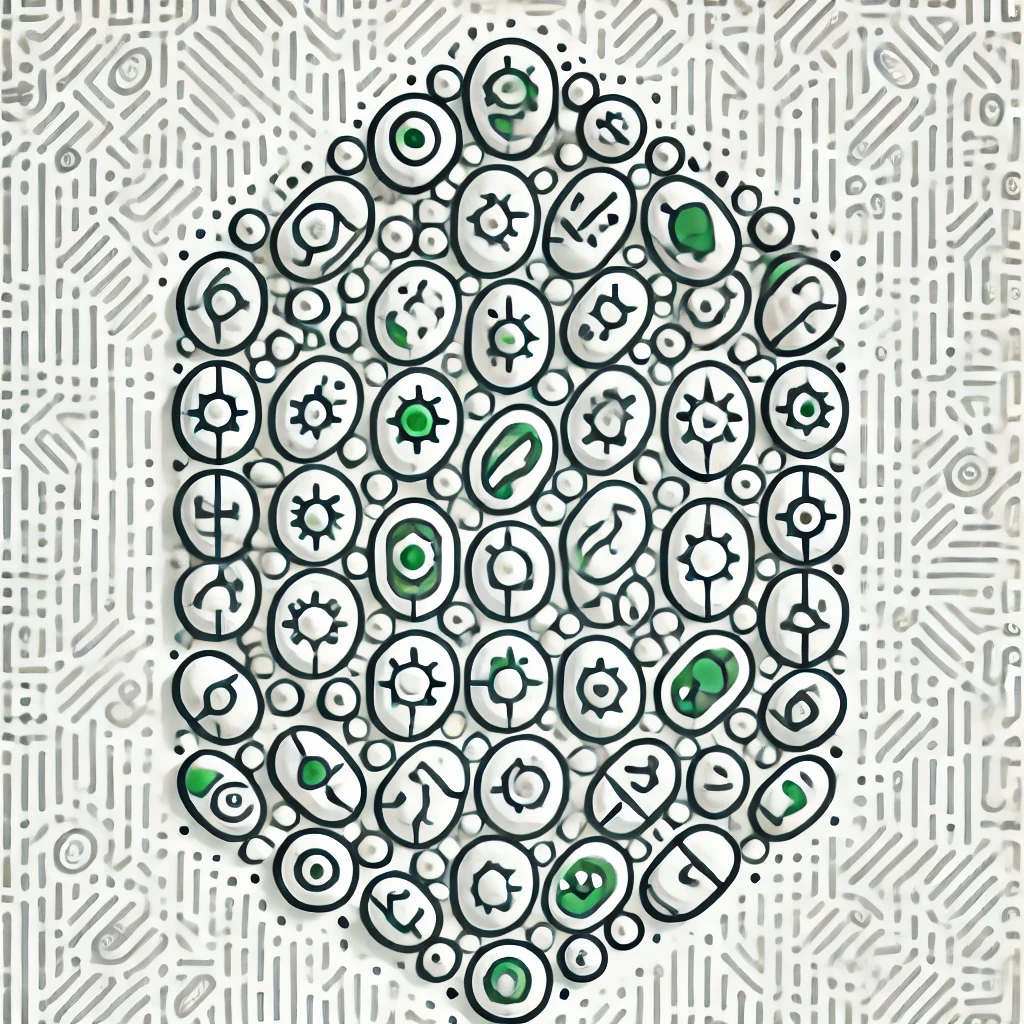
Causal Bayesian Networks
Causal Bayesian Networks are mathematical models that represent relationships between variables, showing how one factor can influence another. They use a directed graph where nodes are variables and arrows indicate cause-and-effect connections. These networks help us understand and predict how changes in one part of a system can impact others, based on probabilities. For example, they can model how smoking increases the risk of lung disease, considering other factors like age or genetics. They’re valuable for reasoning under uncertainty and making informed decisions by capturing the probabilistic causal relationships within complex systems.