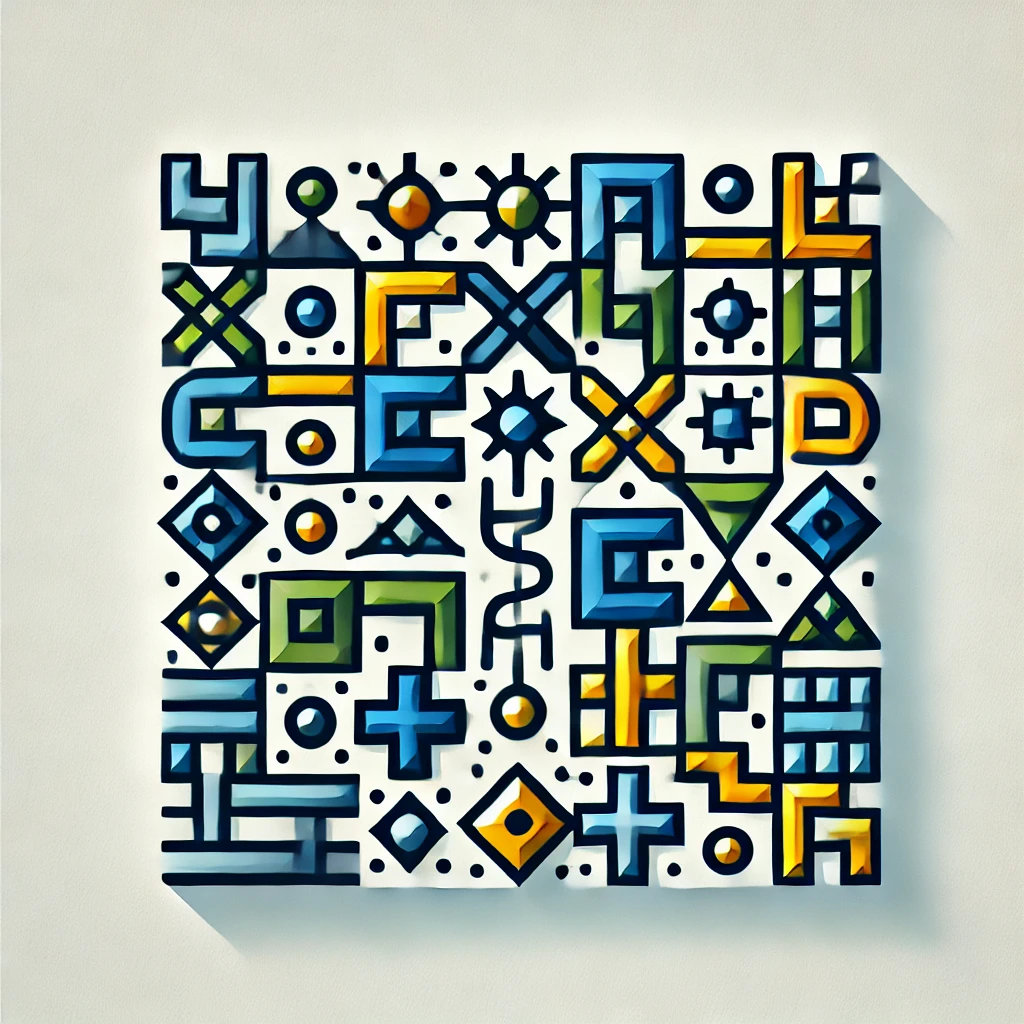
Backward Elimination
Backward elimination is a method used in statistical modeling to identify the most important factors influencing an outcome. Starting with all potential variables included, the process systematically removes the least significant ones—those that have the smallest impact or least statistical evidence of affecting the result. After each removal, the model is re-evaluated to ensure it still accurately represents the data. This process continues until only the most relevant variables remain, making the model simpler without sacrificing its predictive power. It's a way to focus on the key factors and improve the model's efficiency.