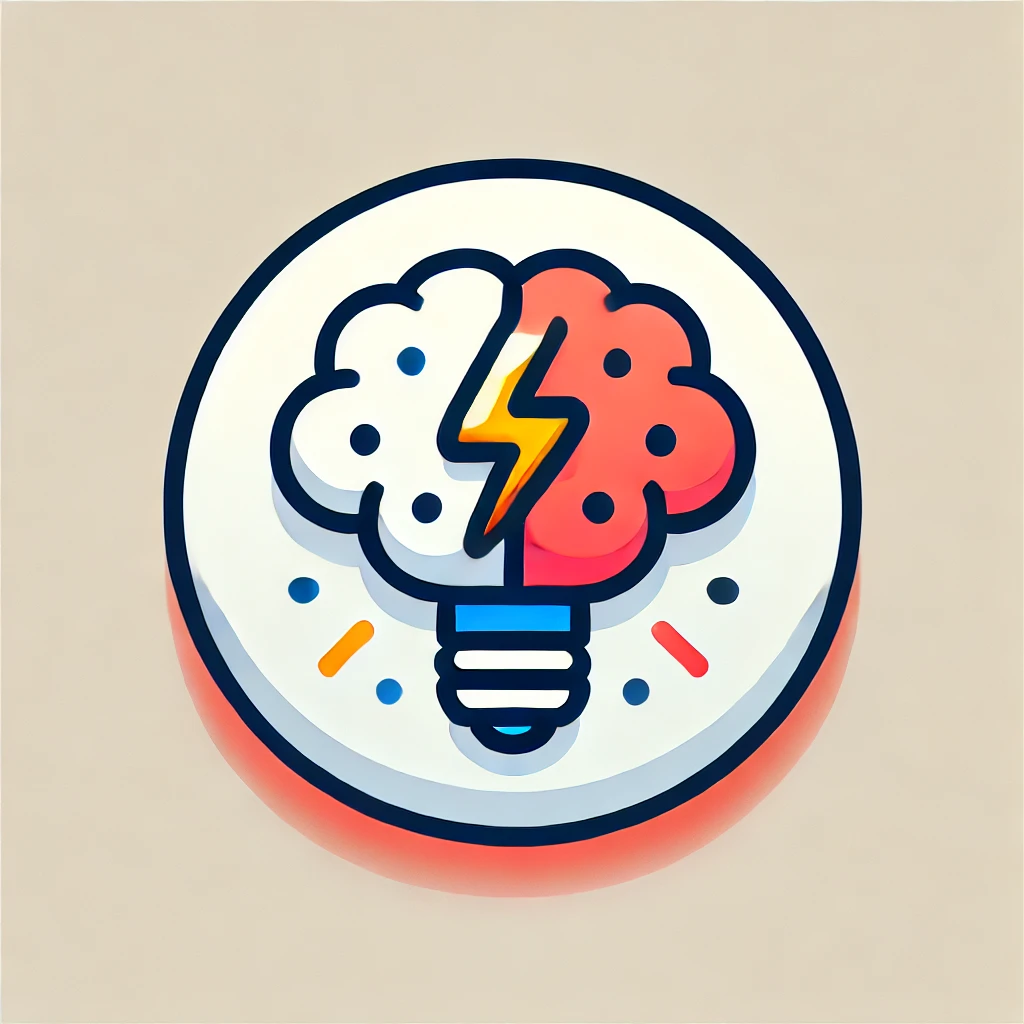
Assumptions of regression
Regression assumptions are conditions that ensure accurate and reliable analysis. They include linearity, meaning the relationship between variables is straight; independence, where data points don’t influence each other; homoscedasticity, meaning the variance of errors is consistent across all levels of the independent variable; normality, where errors are normally distributed; and no multicollinearity, ensuring predictors aren’t too closely related. Meeting these assumptions helps validate the model’s predictions and conclusions. If these conditions aren’t met, the results may be misleading or inaccurate.