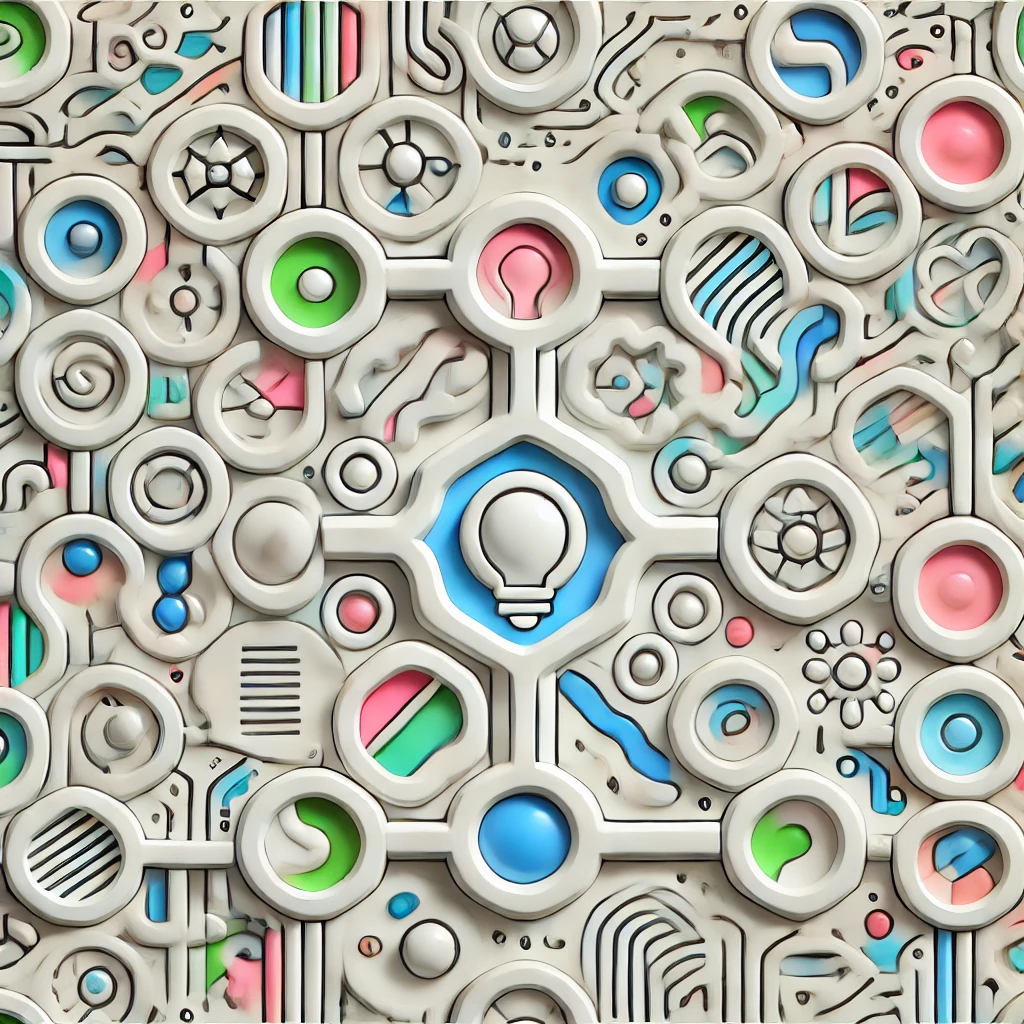
Approximation Spaces
Approximation spaces are a mathematical framework used to analyze how well complex data or functions can be represented using simpler, approximate versions within a specific context. They help quantify the trade-off between accuracy and simplicity, providing ways to measure the closeness of an approximation and the complexity involved. This concept is useful in areas like data compression, signal processing, and machine learning, where it’s important to balance detail with simplicity to efficiently model or analyze information. Overall, approximation spaces help us understand how effectively we can approximate complex systems with manageable, simpler forms.